Draft day derby: The wild world of picking an NFL quarterback
The 2024 NFL draft begins Thursday, April 25, and will be full of intrigue for NFL fans. For teams near the bottom of the standing, the draft represents hope for the future. The right player can turn...
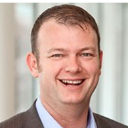