Graph Builder Bar Graph Label Offset
...ffset insert into (qq, (rr)); ); //Make magic happen eval(substitute(expr(seg<<qq), expr(qq), name expr(qq))); );//end of ii for next loop In a previous blog post I described the s...
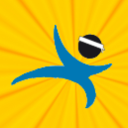
...ffset insert into (qq, (rr)); ); //Make magic happen eval(substitute(expr(seg<<qq), expr(qq), name expr(qq))); );//end of ii for next loop In a previous blog post I described the s...
先週末、世界中がパリオリンピックの選手の活躍に注目していた中、アメリカでは7月の雇用統計が発表されました。今回の雇用統計では失業率が市場予想を上回り、景気後退のシグナルである「サームルール(Sahm Rule)」が点灯されたことが話題となりました。 サームルールとは、元FRBのクローディア・サーム氏が提唱した失業率より計算されます。具体的には、その値(パーセンテージ)が0....
This post is written by a guest blogger. I use the JMP Scripting Index a lot. I even prefer it over the Search introduced in JMP 17 because the Scripting Index isn't context-dependent a...
We were delighted to once again feature independent educator and best-selling author Nick Desbarats, founder of Practical Reporting, on Statistically Speaking. We drew an engaged audience interested ...
...is contributions to peak analysis and peak finding based on his Spectris Add-in, which can be found in the User Community File Exchange. In this blog, @Mark_Bailey and I fill you i...
JMP 18 has a new way to integrate with Python, providing an independent Python environment designed to be used with JMP. In addition, JMP now has a native Python editor and Python packages speci...
Is JMP a Spreadsheet Program? Can JMP work as an FMEA template? It's no secret that FMEA (Failure Modes and Effects Analysis) is typically work that resides in spreadsheets. It's also no secret tha...
多変量データの関係性を調べる際、2次元や3次元程度に次元を削減してデータの関連性を調べる方法として主成分分析(PCA)がよく用いられます。 しかし近年、次元削減の方法としてUMAP、t-SNEという手法が脚光を浴びています。これらは非線形な次元削減手法であり、線形な次元削減方法である主成分分析ではうまくデータ構造を表現できないときでも有効であると言われています。 &nb...
Simple Find and Replace JMP's scripting language, JSL, can store numbers, strings, and other objects in variables. A string assignment might look like this: place = "Cary, North Carolina, ...
Recently I gave an internal JMP talk on Python Tips and Tricks. There's one tip in particular that I think is powerful, so I'd like to share it with you: creating a JSL function that wraps the call t...
Companies in the Consumer-Packaged Goods (CPG) industry need to understand what their customers are saying about their products to improve sales and customer satisfaction. Given the large volume of d...
Esta presentación introduce las nuevas funcionalidades de JMP 18 en español, incluso: Detección de picos Conectores de datos configurables Mejoras la importación de datos en servidor...
Someone in the SAS Communities forum asked this. They wanted to write a script that created a Graph Builder report without knowing in advance how many variables there would be. That is, they w...
...imilar between groups. However, mating tends to be nonrandom to some degree, causing a structure to arise. For example, a barrier like a river can separate two groups of the same species and make it d...
In a previous post titled “Saving graphs tables and reports in JMP,” I shared with you how to copy/paste JMP content into PowerPoint. Now I want to follow up by showing how you can export JMP reports...
...ython packages specific to JMP. This JMP Python environment has enhanced connectivity and interaction with JMP, which means using Python with JMP has never been easier. In this series of blog p...
JMPで分析した結果を組織内でプレゼンテーションする際、JMPのジャーナル機能が役立ちます。 ジャーナルはJMPのファイル形式の一つであり、分析に使ったデータテーブル、レポート、スクリプト、WebのURL、テキストなどを含めることができます。 そのため、プレゼンテーションの内容を一つのジャーナルファイルとして保存しておくと、Power Pointなどを...
...e pasted into publications or reports, including boundaries of significance calculated by the Johnson-Neyman (J-N) technique. The J-N technique calculates values of the moderator at which the simple s...
...otential. Transcending those boundaries has led some cyclists down the unscrupulous path of winning at any cost. This blog looks at past winners – both scrupulous and unscrupulous – to determine if t...
實驗設計 (DOE) 常應用在醫療、生技製藥及高科技製造等產業,做為降低實驗次數及成本的一種方法,在進行實驗設計時有哪些重要原則與步驟?傳統實驗方法中的經典篩選設計與全因子設計的概念與應用有哪些?本篇文章將介紹DOE基本原則、經典篩選設計與全因子設計方法的概念與應用案例。 什麼是實驗設計(DOE) 實驗設計 (DOE) 是透過篩選實驗條件來設計實驗,並且降低外在因子以減少對實...