Hi @giaMSU and @G_M,
Earlier in this thread, I posted a section of script I use all the time when running thousands of training sets in order to just save the R^2, RASE, etc.
As @G_M mentioned, you'll want to go into the tree structure of the report to find out where the values are for you to save. At the very bottom of the script, just before the end of the FOR loop, that is where it pulls the values from the tree structure of the report.
I modified my previous script that automates the NN fitting and included the portion from my previous post that will extract out the fit values (R2 and RMSE). If you want other values, you can add them in as well. The script creates a new data table that has the results of the NN fit for each location ID. I also modified the portion where each data table is generated based on location ID so that the data table has it's own unique identifier based on the location ID, although I just close each data table in the end anyway; in principle you can keep them all open by commenting out the close command at the end of the FOR loop, but then you'll have a massive amount of data tables open.
Before implementing the code, I highly suggest that you read through the comments and understand what the script is doing. Once you become familiar with scripting, it will change your JMP life forever!
On a side note, do you really need to always have the same random seed? I recommend leaving it blank for the fit so that you really get randomness with the fit, which can make it more robust. Also, you have enough observations in your data that you can create a validation column with a training, validation, (and test) set so you can actually find out which of the models is actually the best at prediction. You can stratify this validation column based on your :Y column ("SoilMoisture(kg.m-2) or (mm)"). You can automate this as well and have it in the portion where it creates each of the data tables after summarizing on :ID.
Running the below script on your data table, I get the following output:
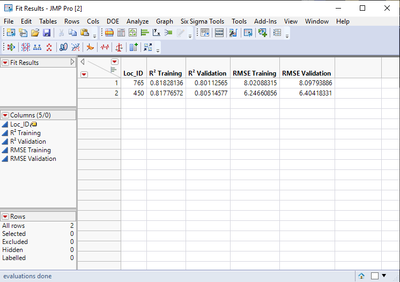
Names Default To Here( 1 ); //keeps variables local, not global
dt = Data Table( "Subset of 765-450" ); //defines your starting data table
Summarize( locs = BY( dt:ID ) ); //creates a list of the different location IDs
//This FOR loop creates all the different data tables based on location ID and also names them based on location ID
For( j = 1, j <= N Items( locs ), j++,
file = dt << Select Where( locs[j] == Char( :ID ) ) << Subset( file, Output Table Name( "Model Subject File Location ID " || locs[j] ) )
);
//this portion of the code generates the list of data tables that will be used to train the models for each location.
dt_list = {};
one_name = {};
For( m = 1, m <= N Table(), m++,
dt_temp = Data Table( m );
one_name = dt_temp << GetName;
If( Starts With( one_name, "Model" ),
Insert Into( dt_list, one_name )
);
);
//this portion of the code creates the fit result data table that will record the statistics for each fit, to be reviewed later. Also adds the label column property to the Loc_ID column.
dt_fit_results = New Table( "Fit Results", Add Rows( N Items( dt_list ) ), New Column( "Loc_ID", "Continuous" ) );
dt_fit_results << New Column( "R² Training" );
dt_fit_results << New Column( "R² Validation" );
dt_fit_results << New Column( "RMSE Training" );
dt_fit_results << New Column( "RMSE Validation" );
dt_fit_results:Loc_ID << Label;
//this FOR loop makes sure that each row of the fit result table also includes the location ID
For( l = 1, l <= N Items( dt_list ), l++,
dt_fit_results:Loc_ID[l] = dt_list[l]:ID[l]
);
//this FOR loop is the fitting routine for each location data table.
For( i = 1, i <= N Items( dt_list ), i++,
NN = Data Table( dt_list[i] ) << Neural(
Y( :Name( "SoilMoisture(kg.m-2) or (mm)" ) ),
X(
:Name( "Tmax(K)" ),
:Name( "Tmin(K)" ),
:Name( "Precip(mm)" ),
:LAI_hv,
:LAI_lv,
:Name( "Winspeed(m/s)" ),
:Name( "Evatransporation(kgm-2s-1)" ),
:LandCover,
:USDASoilClass,
:Forecast_Albedo
),
Informative Missing( 0 ),
Transform Covariates( 1 ),
Validation Method( "KFold", 7 ),
Set Random Seed( ), //I took away the random seed, so your fits will be different from mine.
Fit(
NTanH( 1 ),
NLinear( 1 ),
NGaussian( 1 ),
NTanH2( 3 ),
NLinear2( 0 ),
NGaussian2( 3 ),
Transform Covariates( 1 ),
Robust Fit( 1 ),
Penalty Method( "Absolute" ),
Number of Tours( 1 ) //I only did one tour just for proof of concept, so you'll want to change this
)
);
NNReport = NN << Report;
//These next lines of code are what pull the actual values from the fit report and saves them to the fit results data table.
Train = NNreport["Training"][Number Col Box( 1 )] << Get;
Valid = NNreport["Validation"][Number Col Box( 1 )] << Get;
dt_fit_results:Name( "R² Training" )[i] = Train[1];
dt_fit_results:Name( "RMSE Training" )[i] = Train[2];
dt_fit_results:Name( "R² Validation" )[i] = Valid[1];
dt_fit_results:Name( "RMSE Validation" )[i] = valid[2];
Close( dt_list[i], no save );
);
Good luck!,
DS