You're right @andrewtkarl, the simple approach may work only if all mixture factors have a lower bound at 0, else this might change the lower bound. You can however change the low and high limits of any mixture factors in the Factors panel of the Space-Filling design or use the constraints to get to the desired situation :
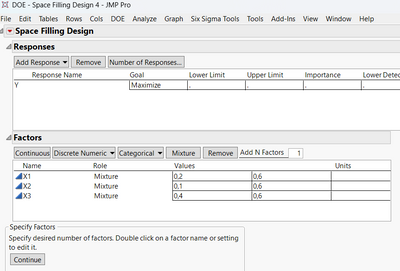
If this doesn't work, try reloading the platform or you can use the dummy factor trick, with a deletion of this dummy variable after :
When creating a dummy factor to solve the problem, you can then proceed to the design creation, but before clicking on "Make Table", go "Back", delete the dummy factor, and very strangely, JMP keeps the high limit of 0,601 and provides a more appropriate design :
Settings with a dummy factor :
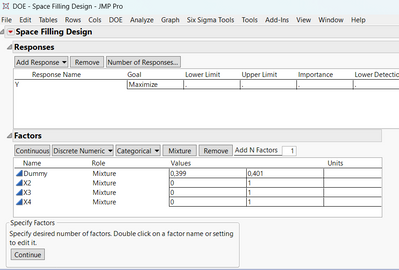
After going back and deleting the dummy factor, JMP keeps the high limit :
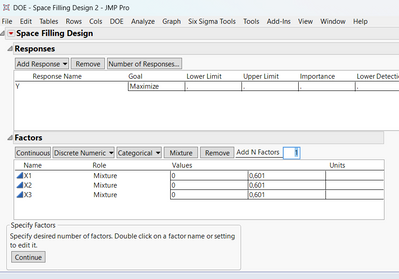
Ternary plot of the design :
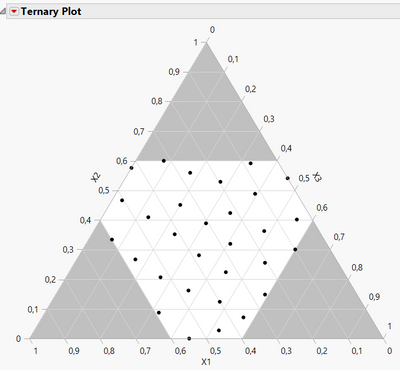
When comparing the distributions of mixture factors with the two methods :
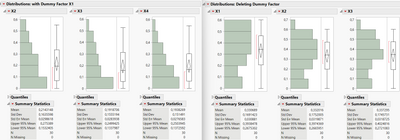
We can see that keeping a dummy factor tends to bias the distributions of other factors towards lower values, whereas deleting the dummy factor and creating the design while keeping the high limits (or simply enter the high limits at the beginning) enable to have a better repartition of the points.
This is also visible with the "discrepancy" values of the two designs (around 1,8 when deleting the dummy factor or with the high limits setting against 3,2 when dummy factor is kept) which also shows that deleting the dummy factor (or directly entering high limits) provides a relatively better design (lower discrepancy is better when comparing two space-filling design).
Related posts : Discrepancy measure for space filling design and https://community.jmp.com/t5/Discussions/Space-Filling-Design-Output/m-p/530412/highlight/true#M7536...
I hope this may help,
Victor GUILLER
Scientific Expertise Engineer
L'Oréal - Data & Analytics