Walker, Biter, The Governor, Negan, JSS, Terminus – if these words raise your blood pressure like they do mine, then you’re probably just as big of a fan of the TV show The Walking Dead as I am.
And if you’re a fan of the show but haven’t made it through season seven yet, then now would be a good time to stop reading this post because there will be spoilers. You've been warned!
The Walking Dead is a TV adaptation of a popular comic book series about sheriff deputy Rick Grimes leading a group of survivors through the zombie apocalypse. While the zombies cause plenty of problems, we quickly learn that rival groups of survivors are the biggest threat to the well-being of Rick and his entourage.
With the eighth season of the show about to start, I got excited to find and analyze some data related to The Walking Dead. As I do anytime I want to learn about something, I headed over to Wikipedia and skimmed over the entry for the show. Using the Internet Open feature in JMP and a handful of table merges, I was able to put together a data set about The Walking Dead without much effort. My data set includes information like number of viewers, writer, director, and a short description (a paragraph or so) for each episode. The figure below gives you an idea of what my final table looks like:
A sample of what the data look like
*****Spoiler alert! This is your last chance to stop reading before there are serious spoilers!*****
The first thing I wanted to look at was a summary of the episode descriptions. The Text Explorer platform was added in JMP 13, and it has quickly become one of my favorite parts of JMP. Text Explorer is a great way to quickly start exploring your unstructured text data. After feeding the description of each episode into Text Explorer, the first thing I like to do is look at the list of most common terms and phrases.
A list of common words and phrases in episode descriptions
The list of terms shouldn’t be too surprising for fans of the show. It is more or less a who’s who of characters in the show. Rick is Rick Grimes, the main character of the show. We see some of the other main characters like Darryl, Carol, the Governor, and Michonne. “Walker” is the term that many of the characters use to refer to zombies, so no surprise that it is near the top of the list.
The list of popular phrases reminds us of some of the major storylines in the show. Rick and his group of survivors struggle with Negan and the Saviors, encounter hordes (and herds!) of walkers, and try to protect their compound in Alexandria. We’re also reminded of the power struggle between Rick and Shane and the romance between Glenn and Maggie. As we can see here, even just looking at the list of common terms and phrases can give us a lot of information about the descriptions of each episode.
Text Explorer provides another useful tool for looking for trends in our text data – Topic Analysis. My colleague Laura provided a nice explanation of topic analysis in a recent blog post. Looking at the first 10 topics contained in the descriptions of Walking Dead episodes, we can clearly make out a few of the major storylines that have unfolded so far. The first topic seems to come from the third season, where Rick and his group are inhabiting a prison and have to defend themselves against the nearby town of Woodbury led by The Governor. After the prison is destroyed, Rick and his group are split up and eventually reconvene in Terminus, which we see in Topic 5. We could go through topic by topic (and I encourage you to do so in the comments!), but I think that it is sufficient to say that as both a statistician and a fan of the show, I thought it was cool how well the topic analysis was able to recover the story arcs.
Topic Analysis of episode descriptions
Now if you’re a fan of the show, you know that season seven of The Walking Dead got started off with a serious shock: Super-villain Negan killed fan-favorites Abraham and Glenn. The season premiere seemed to rattle and upset a lot of fans, so I wondered if people stopped watching the show as a result. The data that I downloaded from Wikipedia includes the number of viewers, so I’m ready to fit a model and look at how viewership changes over time.
Now what does the number of viewers mean in the days of DVR and streaming? I’m not so sure, but it is a (crude) measure of the show’s popularity. If we plot the number of viewers against time, it sure looks like some fans tuned out after what happened to Glenn and Abraham. And this story suggests that the season didn't get much better after the premiere, as the list of least popular episodes contains a good bit of season seven.
Figure 1: Number of viewers for each episode of The Walking Dead
To learn about the show's viewership, I fit a change-point type model with covariates similar to what I did in a previous post about LeBron James. Here I’m using the Lasso in the Generalized Regression platform in JMP Pro to fit a model that will accommodate a moving average as well as other effects like who wrote the episode, who directed the episode, and outliers. It doesn’t seem like knowing the writer or director of an episode would impact a viewer’s decision to watch or not to watch an episode, but I certainly wouldn’t rule it out. Fans of the show can be pretty dedicated, or at least dedicated enough to watch The Talking Dead – a live recap show that follows each episode.
So what did our model tell us? For one, it confirmed that the writer and director didn’t really seem to impact the number of viewers. It also suggests that season premieres tend to provide a boost of almost a million extra viewers. This shouldn’t be surprising because the show always does a good job leaving us hanging in between seasons and building tension for the premiere. Similarly, season finales also provide a bump of about a million extra viewers. There are also some outlier episodes like “The Day Will Come When You Won’t Be," the season seven premiere. Again, not too surprising. The season six finale set up Negan doing something drastic to Rick’s group, and there was serious anticipation built up for “The Day Will Come when You Won’t Be.” Below is a summary of the biggest outlier episodes and how many extra (or fewer) viewers in millions watched than expected.
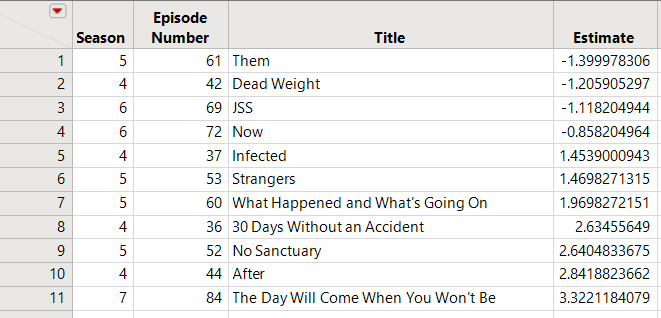
But now onto the big question: Did viewership drop after Negan killed Glenn and Abraham? Once we adjust for outliers, directors, and the other covariates in our model, we’re left with an underlying mean function that we see in Figure 2. The plot of the raw data in Figure 1 is too noisy to draw any conclusions about what is happening, but the trend paints a much more clear picture. This plot suggests that about 400,000 viewers stopped tuning in immediately after the premiere of season seven. And within the next five episodes, the number of viewers had fallen by about 2 million.
Figure 2: Estimated trend function for the number of viewers
Of course, correlation does not imply causation, so we can’t say that the departure of Abraham and Glenn caused people to stop watching (however, this story sure suggests a causal relationship). Maybe the show was already on the slide? Our trend function suggests that the number of viewers peaked between episodes 50 and 68, or late season four through the premiere of season six. I consider that to be a strong stretch in the series (except “Slabtown” of course, that episode put me to sleep quick); it took Rick and his crew from Terminus to Alexandria. But then our trend steadily drops by almost 1 million viewers over the course of the sixth season. Season six included Glenn’s infamous death fake-out; surely that storyline had to make some fans lose interest (I came close. I really didn't like that).
So with the new season about to start, you can bet that I’ll be watching. It will take more than losing a couple main characters to get me to give up on the show. After all, I've already lost almost all of my favorite characters on the show: Shane, Tyreese, Abraham. But I have one more who is still standing strong. Keep hanging in there Carol!