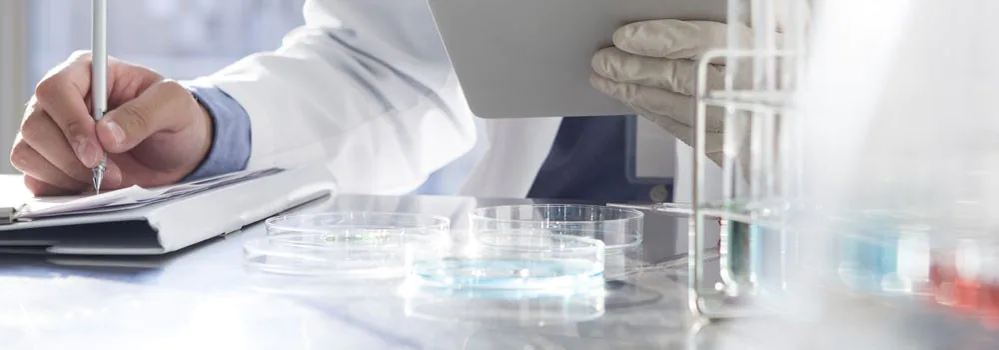
Unlock the Power of DOE
Lately, our team has noticed an uptick in customer requests for guidance on how design of experiments (DOE) can support their product and process development projects, particularly from scientists and engineers that are new to designing experiments. DOE, however, is not a one-size-fits-all process, and crafting a designed experiment involves several factors, including budget, timeline, variable types, and experimental goals. While this versatility is one of the strengths of DOE, those new to DOE might initially feel overwhelmed by the various options and considerations.
In response, we've created a six-part series that aims to provide an introduction to fundamental DOE concepts for those new to DOE, while also presenting intermediate and advanced topics for those who may already be familiar with DOE.
We've named this series "Unlock the Power of DOE" because we believe that DOE is the most powerful tool that scientists and engineers have available to learn about their products and processes. Our goal over these six presentations is to empower JMP users to harness the full potential of this incredibly powerful tool.
Presentations in this series
Session 1: What is Design of Experiments? Click here to watch
Join us to learn about the fundamentals of statistical design of experiments (DOE). This session shows how this experimental method differs from traditional methods and how it enables scientists and engineers to maximize the return on investment in their experiment.
Session 2: Getting Started with Easy DOE Click here to watch
New to DOE? No problem! Easy DOE is a guided platform to get you started. Learn how to design and analyze an experiment all in a single guided process. Once you are comfortable with the basics of DOE, learn how to seamlessly transition to standard DOE approaches.
Session 3: Designing Optimal Experiments with Modern DOE Methods Click here to watch
Modern DOE methods allow you to create an experiment that fits your system. Learn how to overcome the limitations of traditional DOE by using the Custom Design platform. After creating the design, use different tools to explore and compare different designs to make sure you are creating the best option to support your goals.
Session 4: Formulation Optimization with Mixture DOE Click here to watch
Paint, salad dressing, soap. What do they have in common? They are all mixtures. To optimize a mixture, you must change the proportions of the ingredients or components while keeping the total mass or volume the same. If this sounds like a system you are working with, join us to learn how to create and analyze mixture DOEs.
Session 5: Doing More with Less Click here to watch
Are you already using a DOE approach in your experiments but want to continue extracting more information with fewer experiments? Join us to learn about advanced screening designs and how to augment DOEs to build upon prior experiments. The Definitive Screening Design (DSD) is an efficient method that provides a platform for reducing the overall number of experiments AND gives additional information about the system. Augmenting a DOE is a methodology that helps you build upon prior experiments. See how you can start with a simple screening design and then build upon it to learn more without starting a new design.
Session 6: Advanced DOE Topics Click here to watch
JMP continues to push the boundaries of what is possible with DOE in new capabilities and platforms. This session focuses on two of those capabilities: functional DOE and self-validating ensemble models (SVEM). Functional data is two-dimensional data, such as a kinetics curve, heat evolution over time, particle size distribution, or a chemical spectrum. A functional DOE allows you to optimize that entire response curve (instead of a single parameter). Self-validating ensemble models (SVEM) allow you to run even fewer experiments in your design through an advanced modeling algorithms.
You must be a registered user to add a comment. If you've already registered, sign in. Otherwise, register and sign in.