In all seriousness, I started this blog series as "5 things," but I thought of a couple more after I pitched the title and didn't want to change it.
If you're just tuning into the series – yes, I can count. And, yes, we are, in fact, on Thing 6 of 5. Also, here's a brief recap of what you missed:
- I'm sharing five things I wish someone had told me on Day 1 using JMP (now six, I guess).
- Thing 1: Always start with a graph.
- Thing 2: The 4-step JMP workflow everyone should know.
- Thing 3: Why the Analyze menu is set up the way it is.
- Thing 4: Don't underestimate the Distribution platform.
- Thing 5: Save. Your. Dang. Work!
And now on to...
The JMP DOE Workflow
If you can remember "The Four M's," you can quickly master the JMP DOE platform, called Custom Designer.Typically, a classical DOE (design of experiments) workflow will look something like this: You'll first determine the objective of the experiment, and then you'll choose the factors and their levels, select the appropriate response and how to measure it, and choose the design. Then you'll run the experiment, collect and analyze the data, and do some confirmation runs to verify the results.
A lot of this procedure is carry-over from the days when DOE was done with pencil and paper. And, to be honest, it really doesn't set DOE practitioners up for success. It's pretty rare to be able to match up the constraints of modern experiments with pre-canned designs from 50 years ago. It's a square peg/round hole situation.
The JMP development team distilled a lot of this work down to four questions and moved the rest over to computer algorithms. For simplicity, I call these four questions "The Four M's." They are:
- What do you want to measure?
- What do you want to move?
- What relationships do you want to model?
- What materials do you have?
In the process of casting the DOE workflow in to these questions, the developers shifted the order of the classical DOE workflow to something that is, in my opinion, more in line with how modern engineers and experimenters generally approach a problem. If you can remember "The Four M's," you can quickly master the JMP DOE platform, called Custom Designer. Let's look at an example using cookies.
1. What do you want to measure?
The process starts with forcing you to think about what the output of the DOE data set is going to be and by extension, do you have measurement tools needed to get the needed data. Anyone who makes things will recognize the importance of this first step: Are your tools ready? Do you know where they all are? Are they in good working order? This first step is the equivalent of the mise in place concept used in cooking. In the case of our cookies, we are just going to pretend that we have a way to guage how awesome our cookies are (by eating each and every one ... for science, of course). But we could substiute friability (how crumbly it is), color of the cookie (how brown it is), density (how puffy it is), its elastic modulus (how much it bends before it breaks) ... whatever you want. The important point here is that we are thinking about what data we are going to collect before anything else.
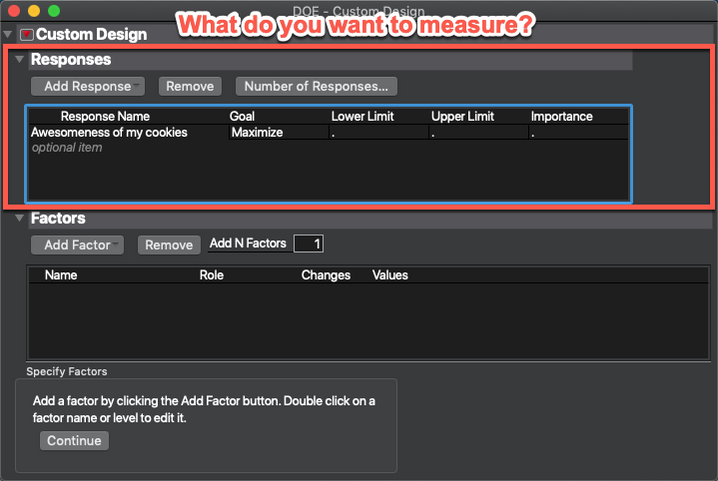
2. What do you want to move?
This question seems fairly straightforward, but there is a wrinkle wrapped up in here that we need to unpack. But first, the workflow asks you to consider the things you consider important. Now, in the case of this example, let's suppose that we found a very old chocolate chip cookie recipe from when ovens were wood fired. The recipe, in general, should be easy to translate into modern units. But, important factors (cooking temperature, cooking time, the form the chocolate should take, and the type of flour) are probably going to be missing. These are the factors that we are going to build our DOE to determine. In the example below, I've added two continuous factors for the cooking time and temperature, and two categorical factors to look at if we should use all purpose flour or bread flour and if the chocolate should be in morsels (what we think of as "chocolate chips") or just a hacked-up bar of choclate (my personal preference – more on that another time).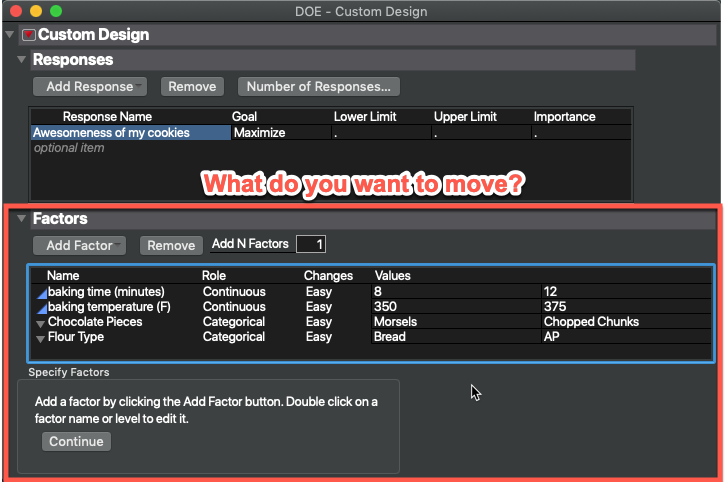
2 (con't). Where can't we go?
Now for that wrinkle. How often do we run into a situation where a classical DOE will propose an experiment that we know is a bad idea? Either we know the experiment is going to fail in an uninformative way or might result in an explosion or some other bad event. In such cases, experimenters will either shrink the design space (reducing the size of the potential signal from that factor) or omit the problematic experiment. Either "solution" compromises the integrity of the DOE. The area below (labeled Define Factor Constraints) where we define the factors allows us to consider these potential problems and design a series of experiments that work around them without compromising the design by using constrained DOEs. There's a lot of information on this in the documentation. 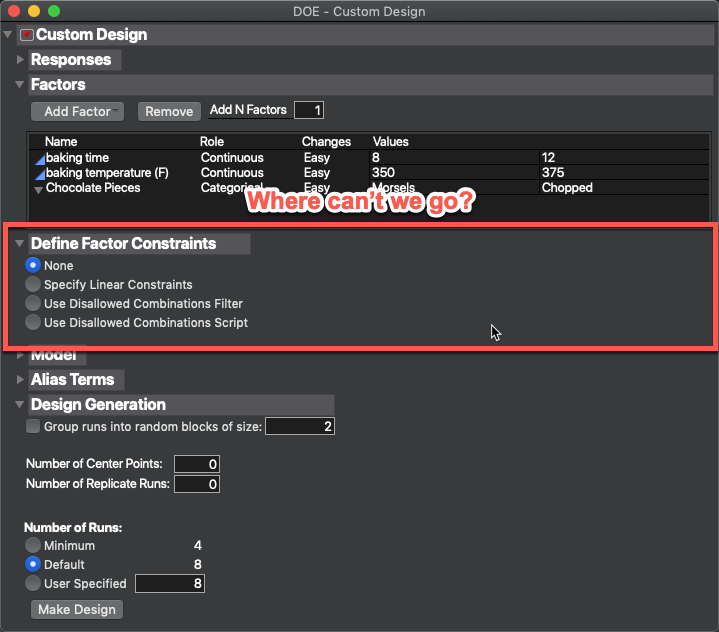
In our case, we know that baking our cookies for too short a time at a low temperature will result in a doughy mess, and cooking for too long a time at high temperature will result in hockey pucks. Graphically, the situation looks like this: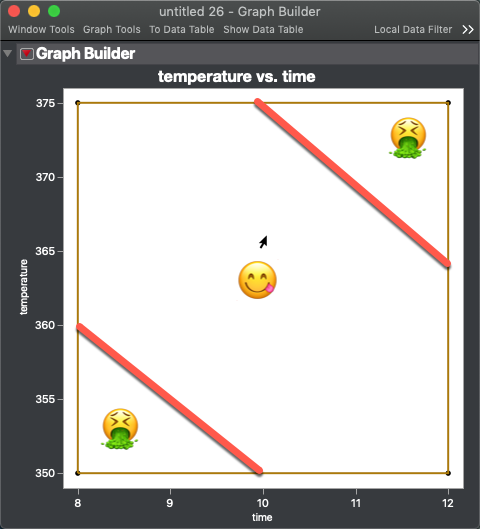
We can define linear constraints (a webinar on how I did the math is found here) to exclude those regions. The setup for this would like the picture below. 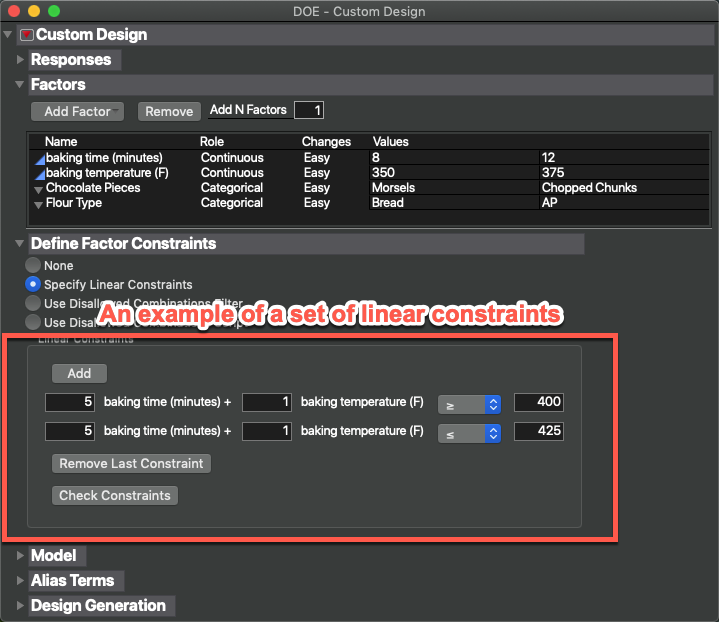
And the resulting DOE would look like this:
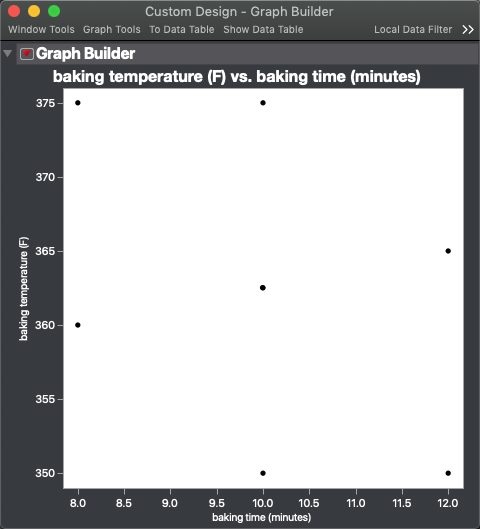
3. What relationships do you want to model?
Classical DOE didn't give you the option to choose what relationships you wanted to test. In Custom Designer, we do. If you only want a specific interaction, why design to test all of them? Another way to think about this is that the Model section allows you to translate your experimental hypotheses into mathematical relationships the algorithms can figure out the best way to test. So, for our cookies, since we've got an old recipe which describes how to make the dough but is missing any information about how to set up the oven for baking, we'll focus on those points. You know that time and temperature are important factors to the observed properties of the cookie. In your head, you could convert that into some hypotheses:
- The baking temperature is important.
- The baking time is important.
- The baking time at a given temperature is important.
- The baking time may have a "sweet spot" somewhere in the range I'm considering.
- The baking temperature may have a "sweet spot" somewhere in the range I'm considering.
In Custom Designer, you would put the following terms in the Model dialog (in the same order as the first list):
- baking temperature
- baking time
- baking time * baking temperature
- baking time * baking time
- baking temperature * baking temperature
It would look like this in the dialog: 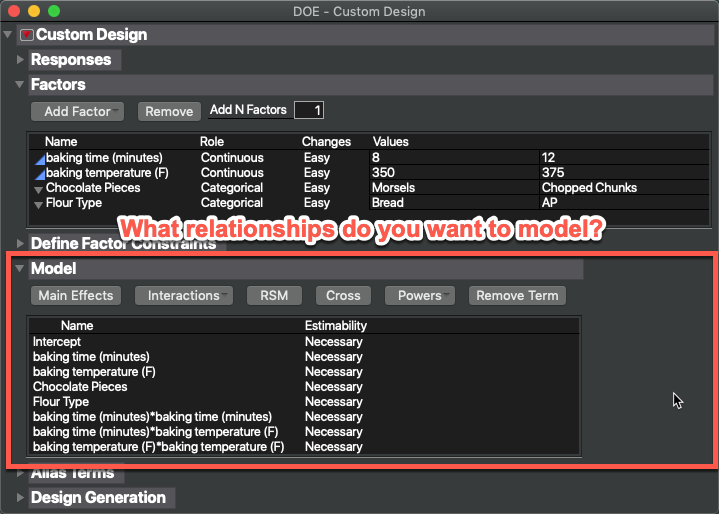
4. What materials do you have?
DOE is a balancing act between experimental needs and real-world constraints (mostly money). The JMP DOE workflow allows experimenters to work with their stakeholders and decision makers to develop a compromise between these opposing forces and understand the trade-offs. The last section of the Custom Design workflow shows the trade-offs between the Model you are asking and the amount of material you need to dedicate to the experiment. If you have more hypotheses, you need more material, and there is a lower limit to the number of experiments you need to perform. 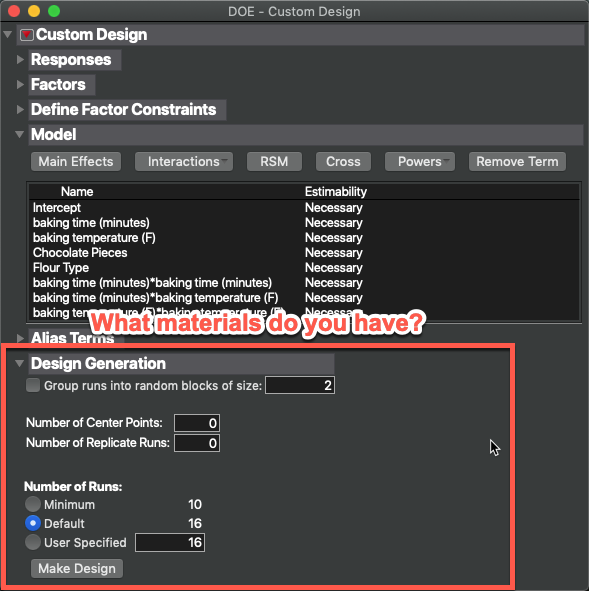
And now, we have finished the true penultimate entry in the series. The next one is it. I promise. And, in my opinion, I have saved the best for last.
You must be a registered user to add a comment. If you've already registered, sign in. Otherwise, register and sign in.