Hi @SimonFuchs,
It's difficult to answer on a very general and broad case, as depending on the number of factors and constraints/specifications, the Custom Design platform may sometimes provide a different design than classical one (like Central Composite Design), but it may also sometimes provide the same design as a classical CCD.
I did a small example with 3 continuous factors and creating 2 designs, one with the "Classical", "Response Surface Design" platform (chosing CCD and axial values of "1", "On Face"), and one with the "Custom Design" platform, and end up with the same CCD designs with 16 runs.
DOE(
Custom Design,
{Add Response( Maximize, "Y", ., ., . ),
Add Factor( Continuous, -1, 1, "X1", 0 ),
Add Factor( Continuous, -1, 1, "X2", 0 ),
Add Factor( Continuous, -1, 1, "X3", 0 ), Set Random Seed( 275411 ),
Number of Starts( 43773 ), Add Term( {1, 0} ), Add Term( {1, 1} ),
Add Term( {2, 1} ), Add Term( {3, 1} ), Add Term( {1, 2} ),
Add Term( {1, 1}, {2, 1} ), Add Term( {2, 2} ), Add Term( {1, 1}, {3, 1} ),
Add Term( {2, 1}, {3, 1} ), Add Term( {3, 2} ), Set Sample Size( 16 ),
Optimality Criterion( "Make I-Optimal Design" ), Simulate Responses( 0 ),
Save X Matrix( 0 ), Make Design}
)
- Script Central Composite Design
DOE(
Response Surface Design,
{Add Response( Maximize, "Y", ., ., . ),
Change Factor Settings( 1, -1, 1, "X1" ),
Change Factor Settings( 2, -1, 1, "X2" ),
Add Factor( Continuous, -1, 1, "X3", 0 ), Set Random Seed( 275411 ),
Make Design( 1 ), Set Axial Choice( 3 ), Center Points( 2 ),
Simulate Responses( 0 ), Save X Matrix( 0 ), Set Run Order( Randomize ),
Make Table}
)
You can compare these two designs, they should be identical.
Could you provide more details to your question ? Do you have a concrete case to illustrate it ?
Maybe you question is more related to the position of star points (on face, rotatable, orthogonal) and its influence on the model (since Custom Design will only create star points "on face" as it is restricted by the range of the factors and can't expand over it) ?
Here is a short Power comparison for the three design types of star points locations: On face (-1/1), Orthogonal (-1,29/1,29) and Rotatable (-1,68/1,68) :
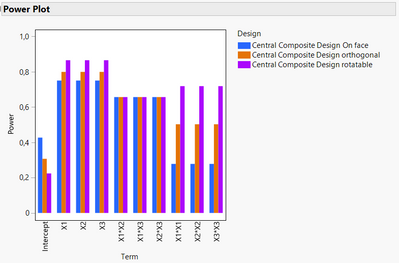
And variance comparison :
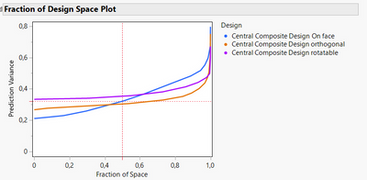
As you can see, the farthest the star points are from the centre of the experimental space :
- The higher the power for main effects and quadratic effects, but the lower the power for the intercept,
- The higher the variance in the centre of the experimental space, but the lower the variances at the border of the experimental space.
I hope you'll find this first answer useful, and depending on your future questions and replies, it may be easier for other members to join the conversation and help you.
Victor GUILLER
Scientific Expertise Engineer
L'Oréal - Data & Analytics