Hi @VarunK,
I won't answer specifically at your questions since @statman did a great job at answering them.
However, one precision about question & answer 1) : If you add the quadratic effect A*A, it won't add a centre point in the design, but (one or) several points in the middle of the range for the factor A (see capture below : on the left, a custom D-optimal design with the term X1*X1 added, so two points (in red) with X1=0 added in the design. On the right, I specifically added centre points (in red) in the custom design).
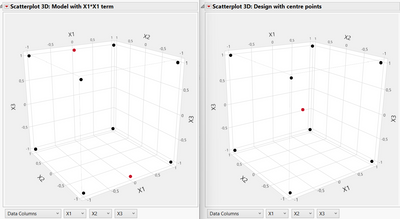
The information from these diverse points is different, so you won't be able to test curvature "in general" by adding specific quadratic terms, you will be able to do it only for the factor(s) for which you added a quadratic term (you have no information or possibility to test for potential curvature effect for factors B and C if you only have points at level 0 for A).
The decision to use centre points or "middle" points (aimed at estimating specific quadratic effects) depends on the objective you have, which design phase you're in (screening / optimization / prediction / robustness), your experimental budget and your domain expertise.
The centre points are commonly used for two main purposes :
- Estimate pure error for the lack-of-fit test, to evaluate if you model seems adequate.
In order to have sufficient power for this lack-of-fit, several centre points (replicates) may need to be done (example here with 3 designs, with 1 to 8 centre points added with quadratic effect X1*X1 power evaluation).
-
Decrease variance prediction in the centre of the experimental space.
This is particularly useful, as your design may often be centered on the initial settings/prior knowledge you have (and factors you want to investigate), so it may be reasonable to have a low prediction variance in this area.
For robustness studies, this is even more important, as the centre points reflect the optimal settings you already have identified and predicted, and the goal is to make sure small variations around this optimum can be handled (or have little to no influence on the outcomes). So you may need the lowest prediction variance possible in the centre of your experimental space when running a robusness study, to make sure small changes are not significant. Example here with the same 3 designs with increasing number of centre points (1, 4 and and their impact on prediction variance :
As you can see, centre points can be helpful in several situations. The decision to add/use them depends on several parameters and also on the model and type of design : optimal design may not require centre points if you already have specified quadratic terms as "Necessary" or "If Possible", but classical designs like Central Composite Design do need them.
You can read some of these ressources for more info :
https://online.stat.psu.edu/stat503/lesson/11/11.2/11.2.1
https://www.statease.com/blog/importance-center-points-central-composite-designs/
I hope this answer will help you,
Victor GUILLER
"It is not unusual for a well-designed experiment to analyze itself" (Box, Hunter and Hunter)