Hi @Bertelsen92,
I think you'll find the explanation from @Mark_Bailey very useful regarding the differences between random and fixed effects : Solved: Re: meaning of random effects p-value in the report - JMP User Community
In your case, 81% of the variance can be imputed to your random effect, your day variable. Having a significant Wald p-value means that the response variance depends on the random effect, so your day variable (the whole plot). But that doesn't mean that your data is "rubbish". You can also first visualize your data and/or your model through different platforms, for example looking at the "Actual by Predicted Plot" available in different modeling platforms might already tell you if you can get a sensible model for your data or not (example from my sample datatable to reproduce the same conditions you have):
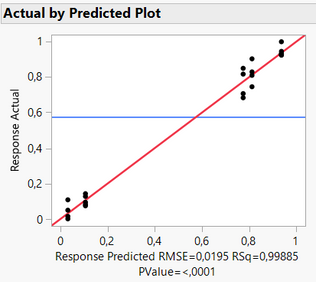
As an example, I added non-constant noise (increasing with the number of the row), and I have a very similar display like you for REML :
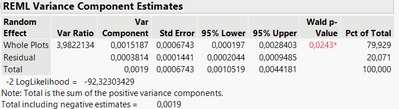
But looking at the fixed effect estimates (and after removing non-significant effects), I was able to detect 2 significant main effects, 1 significant interaction and 1 significant quadratic effect :
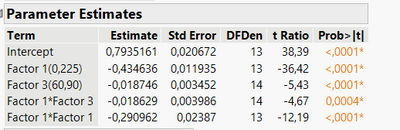
Depending on how large is your noise/variance (compared to the difference between levels' means you want to detect for the different factors/effects), your predictive performance might not be high. But it's worth to look at the Profiler, to have a feeling about how each factors may affect the response.
Additional option for the Profiler: In the red triangle, you can click on "Conditional Predictions" in order to see the model's predictions by fixing the random effects: Prediction Profiler Options (jmp.com) This might be helpful if you want to have a look on the model and the factors' effects by controlling the variance from the random effect.
Example here on the sample data :
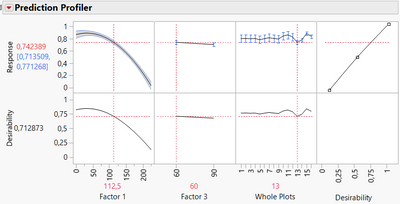
I added my example shown here so that you can reproduce the results I have shown by looking at the script "Model final".
I hope this answer will help you,
Victor GUILLER
Scientific Expertise Engineer
L'Oréal - Data & Analytics