Hi @frankderuyck,
From what I understand from your situation, there may be several options available :
- You can consider your categorical factor as a random effect (or noise), as you're interested on the variance caused by this factor, not by the change on the mean response.
- You can also use the Simulator, to add random noise to a categorical factor and assess how this may change the response distribution.
For the suggestion 1), I used the sample dataset "DOE Example 1" in the JMP sample index (in DoE). I changed the design role property of "Operator" to "Random Block", and run a model with the following effects :
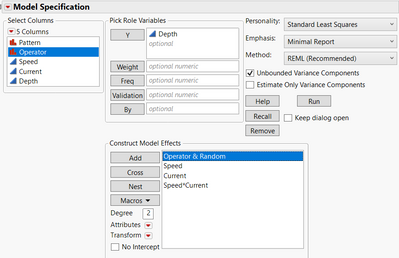
I end up with a Profiler that takes into account the variability introduced by the random effect "Operator" :
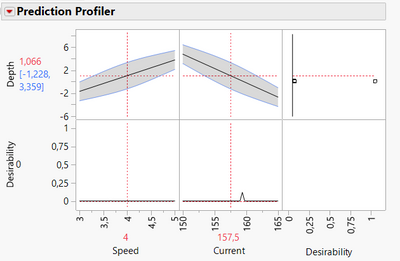
If using the profiler with Operator as a categorical fixed effect (or if set up as random effect, I can click on the red triangle next to the profiler, and make "Operator" factor appears by clicking on "Conditional Predictions"), I can use the Simulator, specify the type of noise (here I choose "Random" with a probability of 50% for each operator) and then click on Simulate :
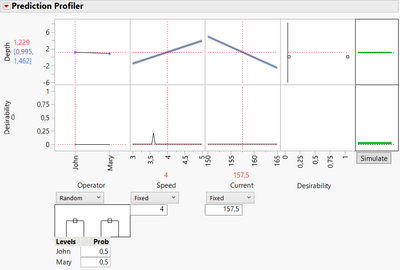
The two approaches are conceptually different (a random effect suppose that these 2 operators are part of a bigger, unknown population which may also impact the variance of the results, whereas the Simulator uses only these 2 possible options to compute the results distribution) and lead to different results, so depending on your topic you may find one more relevant than the other.
I hope this will help you,
Victor GUILLER
Scientific Expertise Engineer
L'Oréal - Data & Analytics