Hi @sam8546,
1) Alpha (also called significance level) is the risk that in a statistical test a null hypothesis will be rejected when it is actually true. This is also known as a type I error, or a false positive. This means in your context the risk you're willing to accept that you detect a statistically significant effect or you obtain a statistically significant model to explain your response, where the null hypothesis is in fact true (so no statistically significant impact of the effects and/or the model to explain the response). The term "risk" refers to the chance or likelihood of making an incorrect decision based on this assumed correct model. A common threshold/value for this risk is 0.05 (so 5% chances of rejecting the null hypothesis when it's actually true), but you can specify it accordingly to your research.
A lower value for alpha (meaning you want to reduce the risk of wrong decisions) reduces the probability of a Type I error, but may increase the probability of a Type II error ("false negative", failing to reject the null hypothesis when there is actually a variation in the factors that is statistically significant at changing the reponse), as the null hypothesis is less likely to be rejected even if it is false.
The confidence level can be deduced from the alpha risk value : CI = 1 - alpha
In your case with a significance level of 0.05, the confidence level is 0,95 (95%).
A possible correct interpretation of this confidence level based on the profiler you show can be : "When CTE_EMC is at 18 and CTE_Subs at 14, I'm 95% confident that the response mean value is between 0,293472 and 1,449778". More infos here : 4.2.1 - Interpreting Confidence Intervals | STAT 200 (psu.edu)
2) That means that you're not able to find a statistically significant relation between the response and your factors based on this model, so you don't have enough evidence that the response is actually influenced by your factors. There may be several options to improve this situation, but here are some :
- Checking if there are any strange/outlier points (doesn't seem to be your case),
- Verifying how noise or other external factors are handled (any external variation/nuisance not controlled ?),
- Collecting more data and create replicates of your experimental runs (new independent runs done at the same factors settings as the previous ones already done)
- Modifying and testing other models that can be supported by your data and design : in case of a curvature (detected by a lack-of-fit test and/or residuals pattern), you may add a quadratic effect for one of your factor (X1.X1 in your model for example).
These are just some options, not an exhaustive list.
3) Very often, a visual analysis can be enough, but you can test it more rigourously if you want/need it. You can save the residuals in your datatable, and using the "Distributions" platform, perform a statistical test to check the normality of the residuals. As an example, here is the goodness of fit test for a normal distribution for the residuals from a model in the DoE datatable sample Battery Data.jmp :
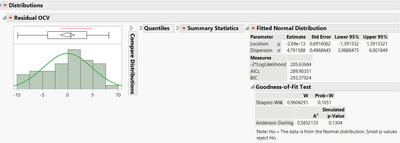
To add a quadratic effect in a model, select a factor in the "Fit Model platform", check that the value 2 is displayed next to "Degree" and click on "Macros", then "Polynomial to Degree" :
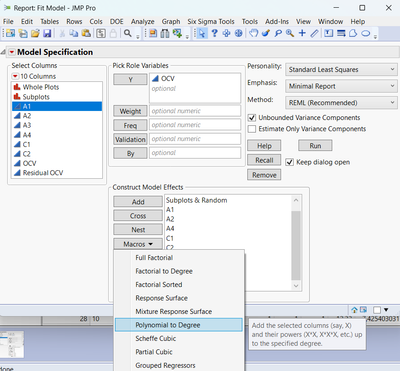
In my example, this will add a new term A1.A1 in the model.
It may be better for you to attend some of the webinar and STIPS courses related to these statistics questions, you'll get a better overview and understanding of what and how to do some statistical analysis and testing, and the various assumptions behind (and how to check it).
Hope this answer will help you,
Victor GUILLER
Scientific Expertise Engineer
L'Oréal - Data & Analytics