Hello everyone,
I've tried to create a sort of mixture design using a script.
The script allows me to have 10 components (a1, a2 - b1, b2, b3, b4 - c1, c2 - d1, d2) and my mixture would consist of only:
a1 or a2 or 0
b1 or b2 or b3 or b4 or 0
c1 and/or c2
d1 or d2 or 0
By selecting the steps, the script provided me with an experimental design table (which I enriched with new experiments and pure components because I had biased coefficients).
For model analysis, I used a second-degree model. I expected it not to work with so few experiments for so many coefficients, but for certain properties, it did work.
I'll illustrate with 2 properties.
For p2, the fit works well; I have a model that fits both observation and prediction.
For p4, the fit works well in observation, but I have huge standard deviation and the prediction performs poorly with a press R-squared < 0.
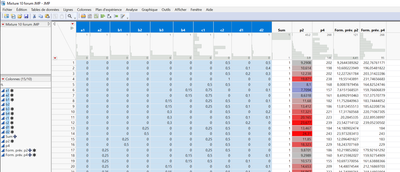
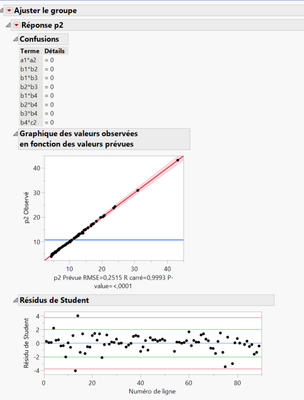
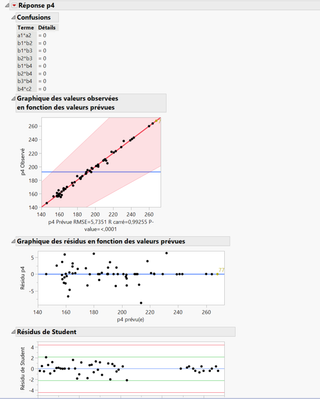
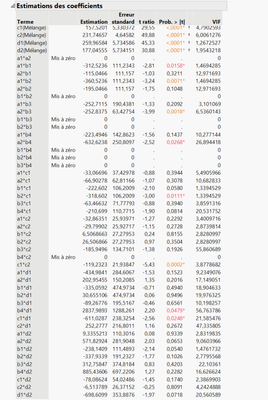
Would you have any feedback or suggestions for improving the quality of my model?
Thank you in advance.