This is based on a recent collaboration with a colleague. He had executed a 3 factor full factorial design with 2 centerpoint runs (a classical, easy design for 3 factors). The results of the analysis of the design showed strong lack of fit, and test for "curvature" (i.e. that there is a quadratic effect missing in the model) was conducted, and that had strong statistical significance. With this design, each quadratic effect cannot be estimated independently, however.
To resolve this, I recommended that he augment his design so that he could estimate the quadratice effect independently. The traditional approach is to add axial runs to the design, and the Augment Design platform allows you to do this easily. In this case, however, he chose the "Augment" option in the platform. He specified that he wanted to group the new runs into a separate block, specifed RSM as the model he wanted to fit, and choose a total number of runs to be 16 (at my recommendation). This will create an augmented design table with the 10 original runs, plus 6 new runs.
The result was unexpected. I had expected a design with axial runs, but instead it gave points six new runs on the mid-points of the 6 of the 8 edges of the design cube. I investigated this a bit further, and I tried some different settings in the platform. I noticed that if I set the Sphere Radius to be less than or greater than 1, then the resulting augmented design would have 6 axial runs added, but if the Sphere Radius=1, then it gave edge midpoint runs. I did not change any of the other settings (like optimality criteria), but I did set the number of starts to be larger (200000). See the tables below and the spinning plots of the designs.
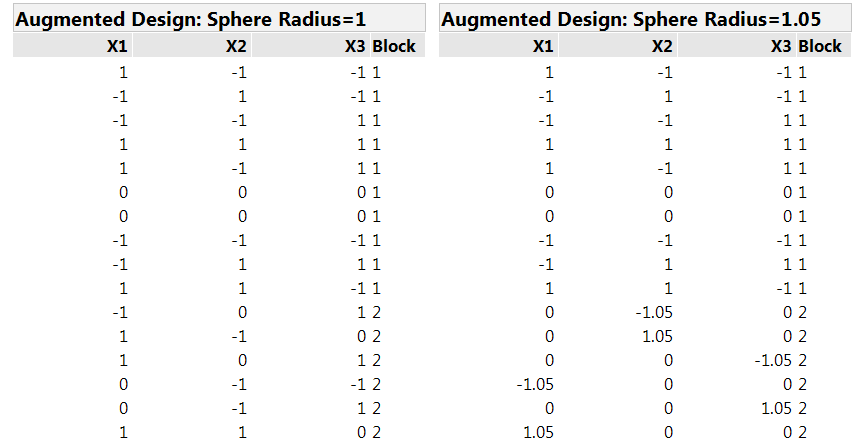
Is this expected?
I did another try using I-optimality as as the optimality criteria, and that gave Axial runs as I expected.
Given all of this, what would be the best practice to use in this situation?