懐中電灯の上に液体の入ったペットボトルを乗せるだけで簡易的なランタンを作成できるのをご存じでしょうか。例えば災害で停電になったとき、懐中電灯だけでは十分な明かりが得られないことがあります。そのとき、水などがはいったペットボトルを懐中電灯の上に置くことによって光が乱反射して明るくなり、広く周りを照らしてくれるのです。
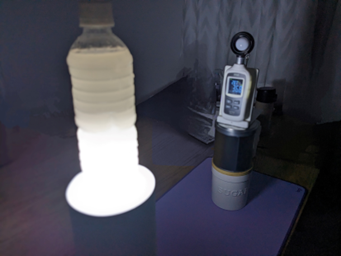
停電時にはできるだけ周りを明るくできるのが望ましいですが、どれぐらい明るくなるかはペットボトルのさまざまな条件によって変動するようです。そこで、実験をしてみましょう。
とはいってもやみくもに実験するわけではありません。実験計画法に基づいて実験をし結論を導き出してみましょう。
「ペットボトルランタン」の明るさ実験
懐中電灯を埋め込んだ土台をつくり、50cmほど離れたところに照度計を設置します。
土台に液体の入ったペットボトルを乗せ、しばらくして照度計の値が安定したところの数値(lux)を読み取ります。
実験の動画を用意しました。
応答は「明るさ(lux)」で、最も大きくする(最大化)することを目的とします。
- 因子はペットボトルにいれてある液体の種類、容量、濃度(常用対数に変換したもの)と、ペットボトルの形状の4つとします。
- 液体の種類として水でも良いのですが、今回はスポーツ飲料、乳酸菌飲料、牛乳の3つを検討することにしました。
因子の一覧
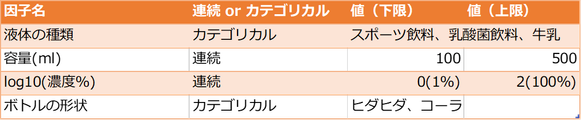
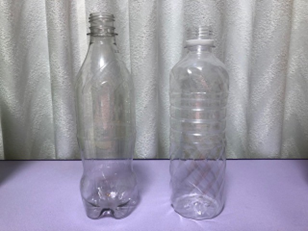
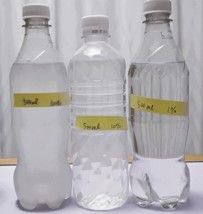
左図:ペットボトルの種類(コーラ、ヒダヒダ)、右図:濃度の違い(100%,10%,1%)
本実験における実験計画法のフロー
まずスクリーニング実験をおこない、4つの因子から重要な因子を抽出します。重要な因子が見つかったらその因子を使って再度、応答曲面をあてはめるための実験をおこない、モデルをあてはめて最も明るくする因子条件を求めていきます(最適化)。
ステップ1: 重要因子のスクリーニング

ステップ2: 応答曲面の作成、最適化

スクリーニング実験
今回のスクリーニング実験では、主効果と、3つの因子「液体の種類」、「容量」、「log10(濃度)」に対する2次の交互作用をスクリーニングの対象とします。(「ボトルの形状」に対する交互作用は考慮しません。)
■カスタム計画による実験計画の作成
JMPの[カスタム計画]を使って、スクリーニング計画を作成していきます。
「モデル」では、主効果と3つの2次交互作用(「液体の種類*容量」、「液体の種類*log10(濃度)」、「容量*log10(濃度)」)を含めます。このとき、実験回数のデフォルト値は18になりますので、ここではこの実験回数で計画を作成します。
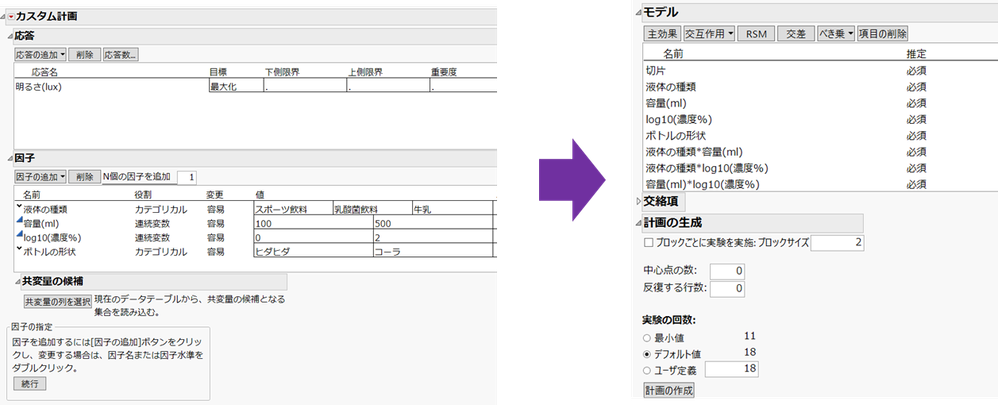
生成された18回の実験計画に基づいて実験をおこない、応答(明るさ)を入力します。
■実験データの可視化
モデルをあてはめる前に、得られた実験データを可視化してみましょう。グラフビルダーを使うと次のような可視化ができます。
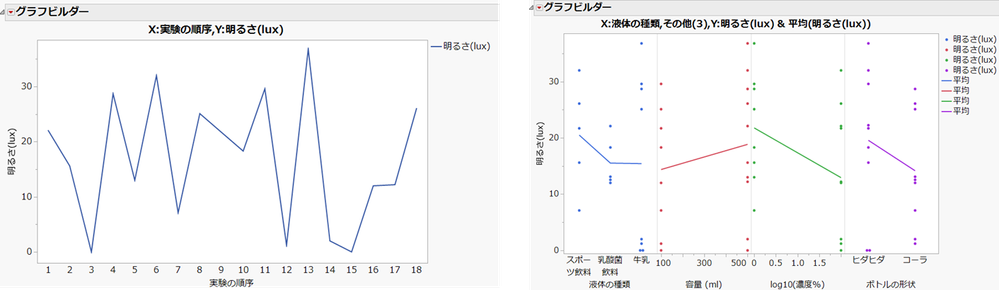
左図:実験順序をX軸、応答をY軸とした折れ線グラフ、実験順序が結果に影響を及ぼしていないかチェックする。
右図:要因効果図、因子をX軸、応答をY軸とした散布図、折れ線グラフ(各水準の平均を結んだもの)、応答と因子の関連性、外れ値の存在などをチェックする。
■分析レポート
データテーブル左上にある「モデル」のスクリプトを実行すると、カスタム計画で指定したモデルがあてはめられます。
- 「予測値と実測値のプロット」を参照すると赤い線(実測値と予測値が一致する領域)から大きく外れた実験点はなく、R2乗は0.96と高い値を示すので、良いあてはめができていることがわかります。
- 「効果の要約」のレポートを参照すると、有意水準5%で有意になっているのは、「液体の種類*log10(濃度)」、「log10(濃度)」、「ボトルの形状」であることがわかります。
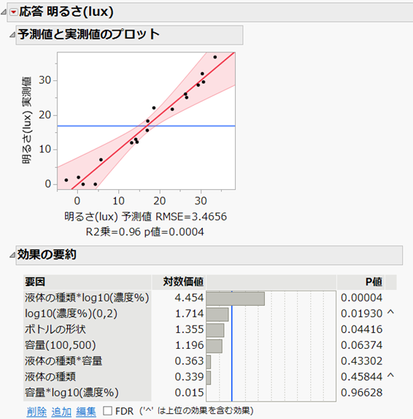
■不要な項を誤差へプーリング
スクリーニング実験では、応答に影響を及ぼす効果をはっきりと見極めるために、あてはめたモデルで有意でない項を除外する(誤差とみなす)ことがあります。JMPではレポート「効果の要約」で削除したい項を選択し、左下の「削除」をクリックすることでその項をモデルから除くことができます。
- p値が最も大きい(p=0.966)交互作用「容量*log10(濃度)」を削除
- 手順1を実施後、p値が最も大きい交互作用は「液体の種類*容量」(p=0.384)なのでこれを削除
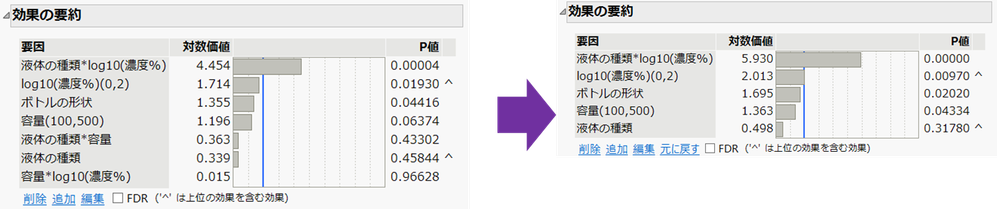
手順1,2では主効果である「液体の種類」はp値が大きいのに削除していません。これは、交互作用である「液体の種類*log10(濃度)」がモデルに含まれているからです。一般にその主効果を含む交互作用がモデルに含まれている場合、その主効果は削除しません。これを効果の階層性といいます。
以下は不要な項を削除したモデルについて考察していくことにします。
■交互作用プロファイル
この例では、液体の種類と濃度の交互作用が高度に有意になっています。この現象は交互作用プロファイルで考察すると良いでしょう。レポート左上の赤い三角ボタンから [因子プロファイル] >[交互作用プロット]で表示できます。
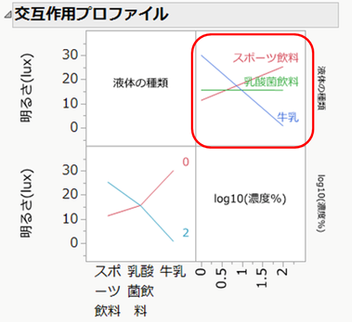
赤枠で囲んだ右上の図をみると、液体の種類と濃度の交互作用効果が顕著に見て取れます。
牛乳の場合は濃度が高いほど明るさは減少傾向にありますが、スポーツ飲料は濃度が高いほど明るさは増加傾向にあります。乳酸菌飲料は、濃度と明るさに関係がないように見えます。
■予測プロファイル
予測プロファイルを使って、応答と因子の関係を考察します。
左にある赤い三角ボタンから、[最適化と満足度] > [満足度の最大化] を選択すると作成したモデルにおいて、応答である明るさの予測値を最も大きくする因子の条件を求めることができます。
明るさを最大にする条件は、ヒダヒダのペットボトルに濃度1%の牛乳を500ml入れたときです。このときの明るさの予測値は約34luxになります。
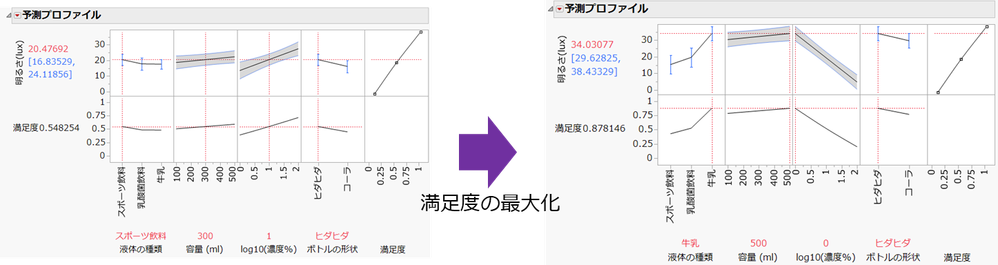
ご存じの通り、牛乳には油脂成分が含まれます。この油脂成分が明るさの要因となっています。昔は油を明かりの燃料として使用していたことからも理解できます。
非常時には、ペットボトルの水に少量の牛乳を入れると非常に明るいランタンができるようです。ただし、牛乳そのもの(濃度100%)を使用してしまうと光を遮ってしまうため、注意が必要です。
スクリーニング実験から最適化実験へ
とはいえ、非常時に牛乳を持っていることはほとんどないでしょう。非常時に備蓄するのは水やスポーツ飲料の方が一般的かもしれません。そのため、次回のPart 2ではスポーツ飲料を対象に、最も明るくするための容量と濃度の条件を調査するための応答曲面モデルを作成してみます。
本実験の参考動画: 懐中電灯をランタンに、一番明るくなる方法は?|防災実験[そなえるTV]YouTube
https://www.youtube.com/watch?v=NbmN2s-Pjz8
by 増川 直裕(JMP Japan)
Naohiro Masukawa - JMP User Community
You must be a registered user to add a comment. If you've already registered, sign in. Otherwise, register and sign in.