The Cross Evaluation tool in JMP Genomics allows for the evaluation of potential crosses using genetic markers and scoring code files. A scoring code is a SAS file containing a predictive model which quantifies the effects of numerous markers on a trait of interest. The scoring code files used in this example were created in last week’s post, Cross Evaluation and Progeny Simulation with JMP Genomics, Part 2: Predictive Modeling for Breeding .... The dataset containing the individuals to be crossed must be in wide format and have biallelic markers which are defined using a numeric format. The dataset that will be used as input for this example was created in the post, Cross Evaluation and Progeny Simulation with JMP Genomics, Part 1: BLUP for Breeding Trial Data. In this week’s example, we will evaluate all possible outcrosses in a set of 309 barley lines with ~3,500 markers each, make selections based on that evaluation, and simulate multiple generations of crossing based on those selections.
- From the Genomics Starter, select Genetics > Breeding Analysis > Cross Evaluation to bring up the dialogue box.
- In the General tab, select barley_impute_imgsas7bdat as the Input SAS Data Set.
- The data set contains markers for each line and 4 quantitative traits, 2 of which we will be selecting for.
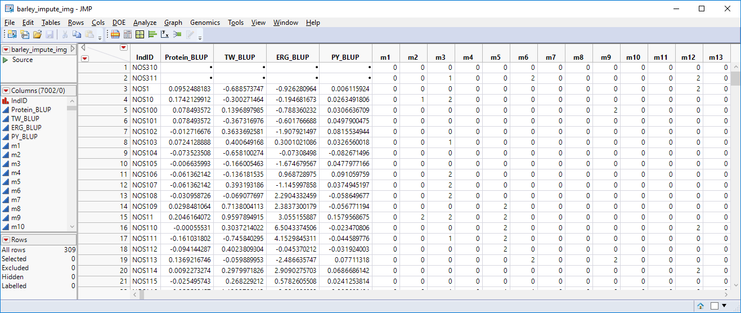
- Select both IndID as the ID Variable.
- Leave the Sex Variable
- Note that values for the Sex Variable must be either “1” and “2” or character strings starting with “M” and “F”.
- Also, note that when a sex variable is specified, no self-crossing can be included in the analysis.
- In the List-Style Specification of Marker Variables box, type m: to specify all columns beginning with the letter “m” as marker variables.
- Navigate to the Folder Containing Scoring Code Files and designate it as such. This will populate the Available Files box with your Scoring Code Files. Select from the list the files corresponding to the traits to analyze and move them to the Scoring Code Files
- A scoring code file is a SAS program file containing a model which includes the effect of each marker on a trait of interest. The file can be previewed using a text viewer.
- Anywhere from one to four of the phenotypes in this data set can be selected for by simply including or excluding the scoring code files for the desired phenotypes. Here, we will select for Ergosterol Content (ERG) and Test Weight (TW).
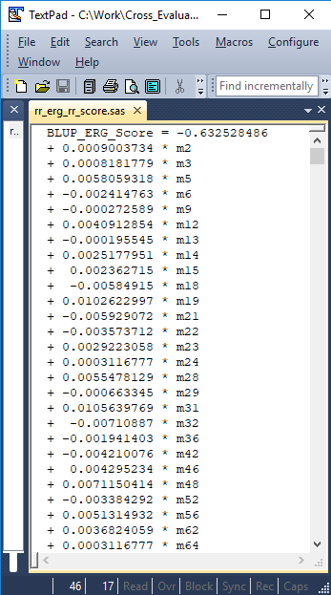
- Select an Output Folder.
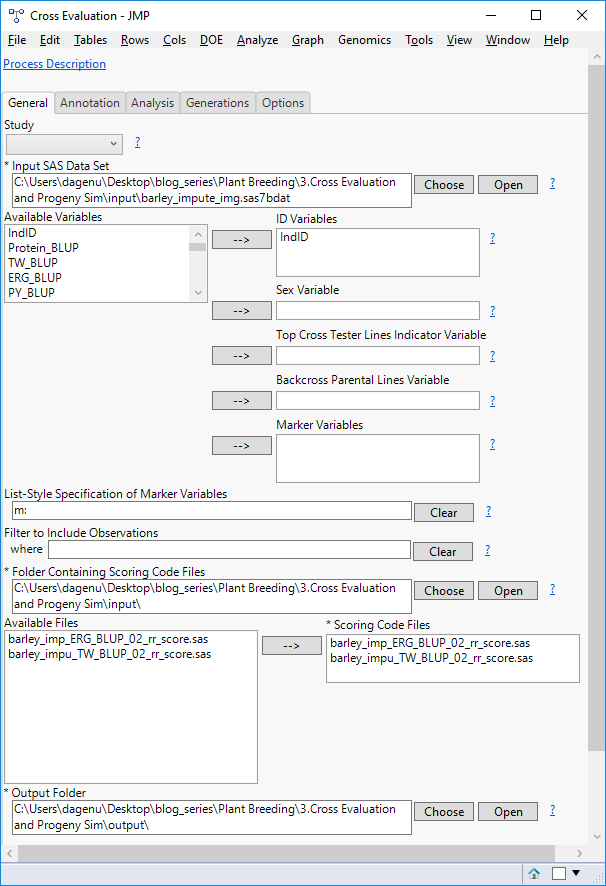
- The Annotation tab can be used to include marker positions and chromosome numbers when an annotation data set is present. Leave the annotation tab blank for this example.
- In the Analysis tab, an option is available to specify a SAS Data Set Indicating Which Crosses to Simulate. If left blank (as in this example), JMP will simulate all possible crosses.
- When a Sex Variable is present and the Maternal Value of the Sex Variable is left blank, JMP will designate either “1” or “F” as the maternal sex variable as a default.
- Select Include outcross only for the Choose the first-generation mating type
- Options for backcrossing (when a backcross parental line variable is specified), progeny simulation using genetic mapping (when an annotation file is present), and including a selection index (a weighted value of the effect of each trait) are also available on the Analysis. Leave these blank for this example.
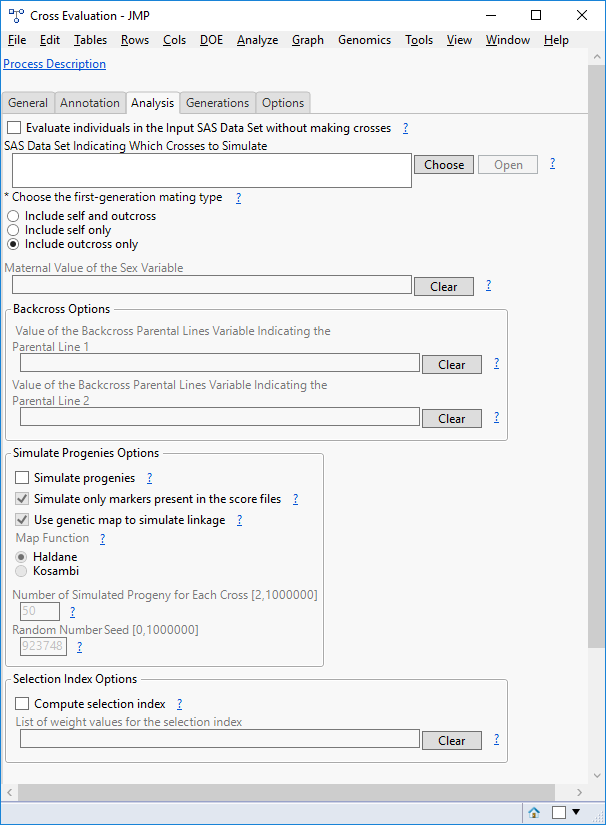
- On the Generations tab, there is an option for simulating multiple generations simultaneously. That is, simulating the progeny of the simulated progeny from the first cross evaluation analysis. This type of analysis takes considerable time and disk space, so it is recommended that it only be done when a set of pre-selected crosses are specified.
- Note that further generations can also be simulated by selecting crosses from the results of this analysis, which will be shown later in this example.
- On the Options tab designate the name barley_crosses for the Output Data Set which will contain the crosses.
- Check the box next to Compress output data set to apply SAS compression to the resulting data set.
- Click Run to begin the analysis.
Cross Evaluation Results
- When the analysis is finished, a results window will appear with a scatterplot matrix showing correlations of the mean, standard deviation, max, min, and range of every simulated cross. Each of the paternal and maternal individuals are listed to the left of the matrix with the number of simulated crosses that they were involved in given in parentheses. Selecting any of the individuals will select all its crosses in the scatterplot matrix.
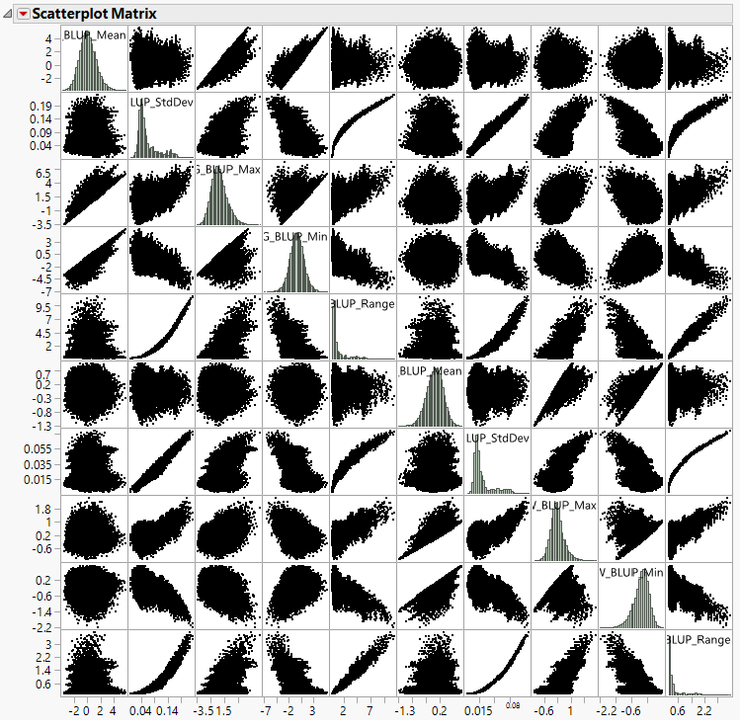
- Clicking View Data on the far left opens a new data set with each of the 47,586 simulated crosses and their phenotypic estimates. Selecting any point in the scatterplot matrix or any individuals from the maternal/paternal ID lists will also select those crosses in the data set.
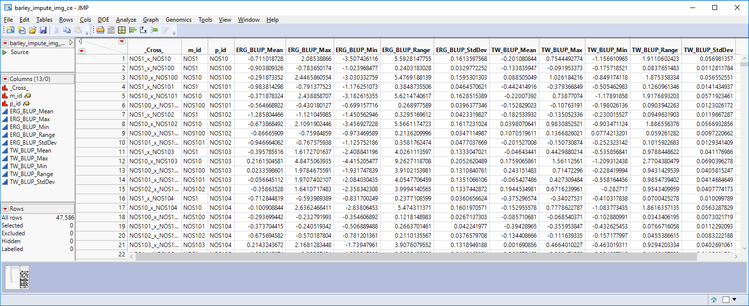
Drill Downs
- To simulate the progeny of any of the resulting crosses, we can select the crosses to include and click the Simulate Progeny box from the Drill Downs. This will bring up a new dialog box identical to the original Cross Evaluation dialogue box. This new Progeny Simulation dialogue box is filled out with the proper settings to evaluate the progeny of any selected crosses from the results of the previous analysis.
- Before making selections for progeny simulation. Click View Data under the Drill Downs menu and look at the distributions of the two traits by selecting Analyze > Distribution.
- Select BLUP_ERG_Mean and BLUP_TW_Mean and move them into the Y, Columns. Then click OK.
- From the distribution tab, percentiles of each trait’s distribution are easily seen. Using the percentiles from the phenotypic distributions, return to the results window and the Data Filter.
- Data filters can be applied to the first generation progeny under the Data Filter. Filtering criteria for each trait can be selected by adjusting the sliders under the trait of interest or clicking the numbers next to each trait and typing the desired cutoff.
- Select only crosses that meet the following criteria:
- 5 percentile for ERG (ergosterol content)
- 90 percentile of TW (test weight)
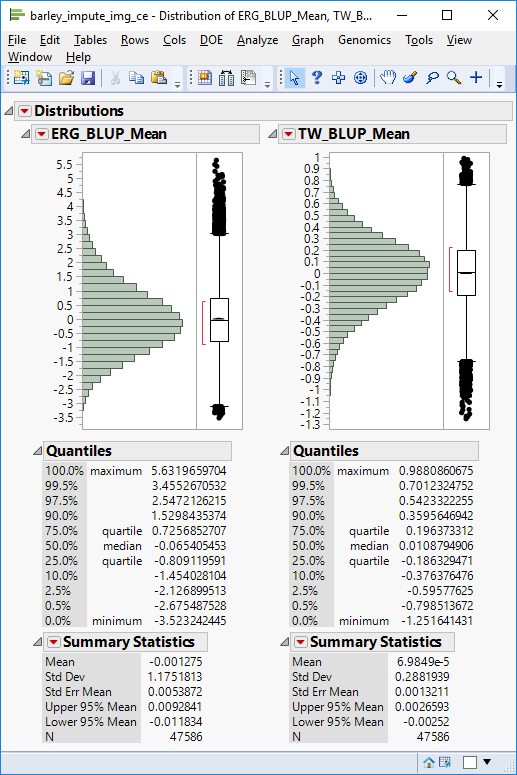
- Filters can be applied using the sliders or by typing the desired cutoff into the appropriate box.
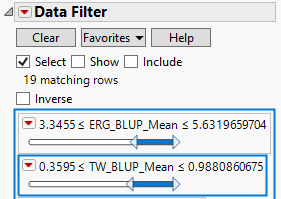
- Once filters are applied, the resulting crosses will be highlighted in the scatterplot matrix.
- When the filters are applied and the desired crosses are selected, click Simulate Progeny in the Drill Downs menu to bring up the progeny simulation dialogue box. A new data table will also appear with a subset of the 19 selected crosses, called selected_crosses.sas7bdat.
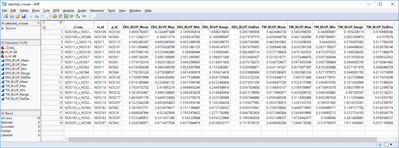
- In the Progeny Simulation dialog box, the General tab (and Annotation tab when applicable) will be completed with information from the first generation simulation.
- On the Analysis tab, the selected_crosses.sas7bdat data set will be entered as the SAS Data Set Indicating Which Crosses to Simulate.
- In the Simulate Progenies Options box, set the Number of Simulated Progeny for Each Cross to
- On the Generations tab, select Simulate multiple generations and select Self as the option for Choose the multi-generation mating type.
- Set the Number of Generations option to 3 to simulate 3 generations of selfing from our selected crosses and click Run to begin the analysis.

Progeny Simulation Results
- When the analysis is finished, a results window will appear with the Simulated Trait Distribution tab showing distributions of each of the simulated trait measurements for each of the three simulated generations. Generation 3 is shown below.
- Scroll to the right to move through the generations note that each generation has 20x more observations than the generation preceding it.
- Scroll down to see scatterplot matrices showing the correlations of the traits for each generation.
- Note that selecting any points on the histograms or scatterplots will select those points on every plot for the corresponding generation. Individuals can also be selected in the Data Filter section to show their performance in each of the plots.
- The Simulated Trait Ordered tab gives heatmaps for each simulated generation of the traits ordering crosses on the x-axis by their performance. The three generations of ergosterol content (ERG) performance are shown below.
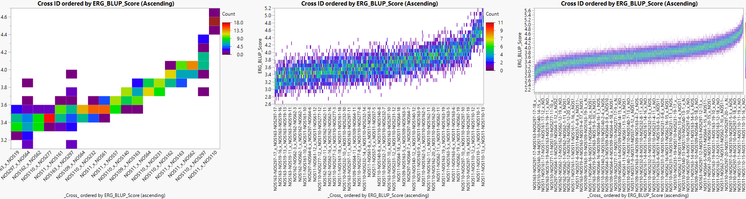
- The Simulated Trait Statistics tab gives similar output to the original cross evaluation output window for the BLUP values of each trait, that is, a correlation matrix and scatterplot matrix for statistics of each trait.
- The Simulated Trait Means tab gives the mean of all simulations for each generation of each trait. The size of the points represents the standard deviation of the population.
- Judging by the trait means, there was not much improvement in the ERG BLUP mean through the first 3 generations.
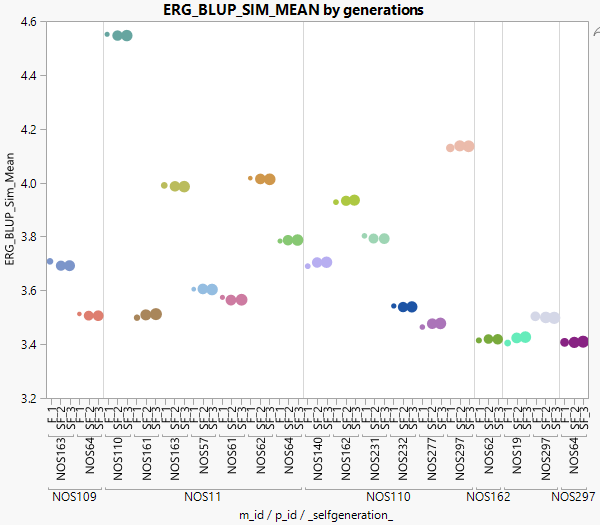
- The Simulated Trait Means Ordered tab displays a plot of each of the 19 crosses in ascending order for performance in each trait.
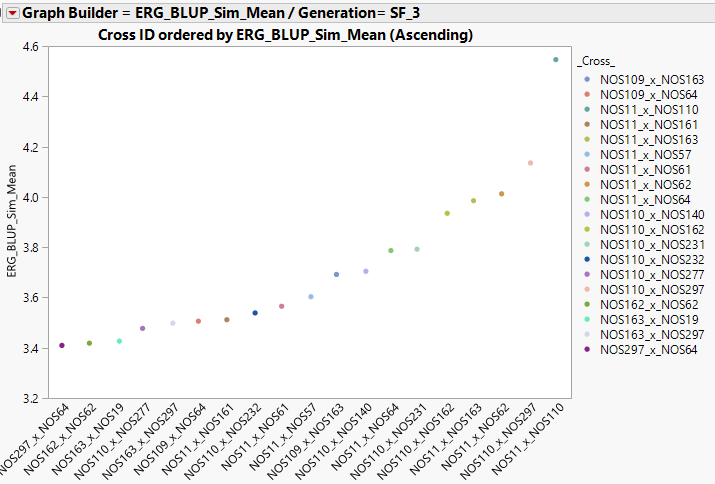
- Further selection and cross evaluation can be done by selecting crosses from the Simulated Trait Distribution charts and clicking Cross Evaluation in the Drill Downs. Data subsets can also be created by filtering in the Data Filter panel or selecting points on any plot and clicking View Data.
Summary
The Cross Evaluation tool in JMP Genomics can quickly analyze all possible crosses from a pedigree data set when combined with a scoring code file. The result is a new data set with statistics surrounding a disease or traits of interest based on marker data. Note that an annotation data set can be included to further incorporate genetic linkage into the analysis. From the output window, crosses of interest can be selected and included in further iterations of progeny simulation allowing for multiple generations of simulated genetic selection
You must be a registered user to add a comment. If you've already registered, sign in. Otherwise, register and sign in.