If you wanted to stick with the mixed model approach with repeated measures, I guess you could do PCA on the mRNA, save the largest impact PCs and then use those in the response role (kinda like what PLS will do). Then do the repeated measures as one might do in Mixed Models or Standard least squares like in this tutorial.
Or put all of the mRNA in response, choose mixed model, set it up as one would for a time based repeated structure and then put treatment in to Fixed Effects (I am probably missing something to add). Then use the red triangle and choose Options for Many Responses. You will get a table for everything instead of a report and that might help, but then there will be some extra work to sort out which mRNA is most affected and other stats for each of the model terms.
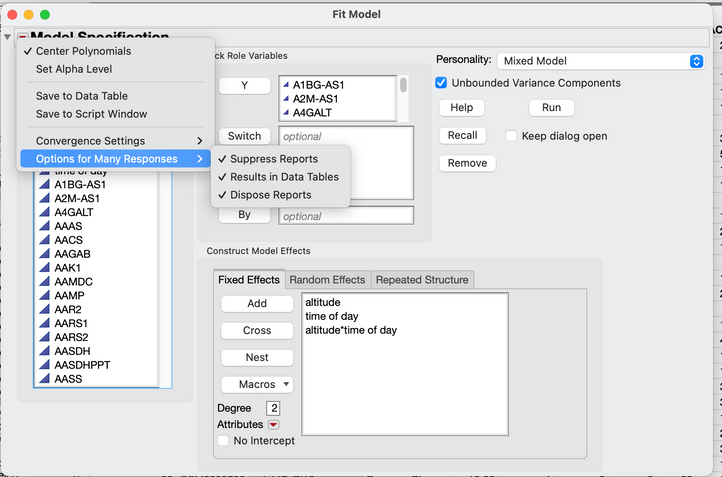
It's a thought anyway. Might be worth it.
Chris Kirchberg, M.S.2
Data Scientist, Life Sciences - Global Technical Enablement
JMP Statistical Discovery, LLC. - Denver, CO
Tel: +1-919-531-9927 ▪ Mobile: +1-303-378-7419 ▪ E-mail: chris.kirchberg@jmp.com
www.jmp.com