Hi @BenGengenbach,
There are still missing informations about the model terms you want to estimate in your model (or I might have skipped this information), but I will try to help you best. I have created a design with the factors description you mentioned, number of whole plots (8)/sub plots (16) and a model with only main effects (48 runs) in order to have more informed and illustrative answers that correspond to your problem.
First, no problem about the fact that "Hard" and "Very hard to change" factors can not vary independently, this is already an option in JMP during design creation when introducing these types of factors (as you mention):
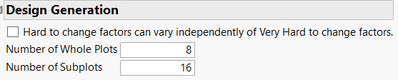
Concerning your questions :
- "Now can we simply run the first half of the split-plot design on day 1 (whole plot 1-4) and the second half on day 2 (whole plot 5-8) without the need to introduce another Day Blocking factor?" : One useful way to look at a randomized block experiment is to consider it as a collection of completely randomized experiments, each run within one of the blocks of the total experiment. Inside each whole plots there is a randomization of the factor levels for easy-to-change factors. Hard-to-change factors levels are grouped in subplots. When looking at the distributions of the factors levels for the first 4 whole plots (or first 8 subplots), you can see that the levels repartition is balanced for all factors :
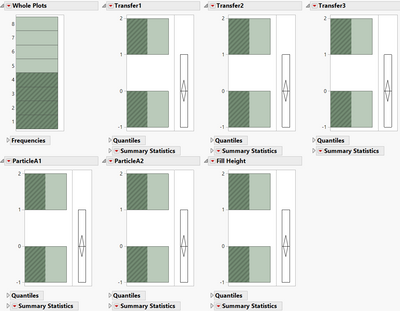
I would have think the same as Phil to add a blocking variable "Day" would help, but in this case whole plots and subplots effects are already treated as random effects (so you can already estimate the variance of your response(s) from whole plots to whole plots and from subplots to subplots), and adding a blocking variable here would mess the structure of whole plots and subplots (as you already show) :
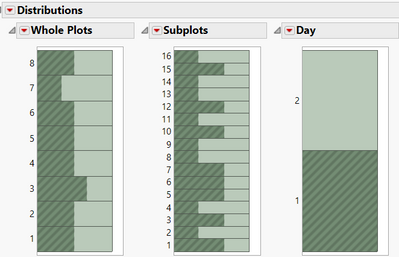
- "Also should anything keep us from analyzing the first half to check if we might already have achieved sufficient precision and only run the second half if the results of day 1 are inconclusive?" : Same answer as Phil, you can absolutely have a look at your first initial results. Just keep in mind that analyzing only half of your results means that you'll have a lower power to detect effects, so a non-significant effect from the analysis of day1 results may become significant when all results are present. Here are screenshots of the power analysis of day1 datatable vs. complete datatable :
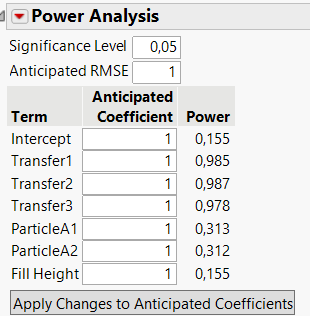
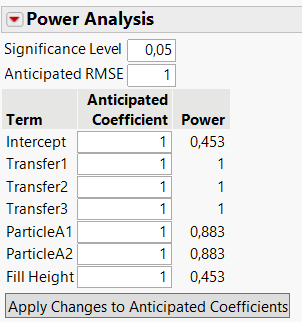
The biggest change for detecting effects can be seen for main effects involving hard/very hard to change factors.
I hope these answers will help you.
Please find attached the datatable used for illustration in my answer.
Victor GUILLER
L'Oréal Data & Analytics
"It is not unusual for a well-designed experiment to analyze itself" (Box, Hunter and Hunter)