Your last comment about testing mortality rates over time changes everything, I think. My example is not relevant. My example is a case where a number of samples or subjects (20) is assigned to each factor level (5). Each level is an independent sample (5x20). The total number of samples or subjects is the sum of the samples at each level (100). I believe that you start with a fixed number of subjects (e.g. 60 total), the agent level is introduced at a fixed level, and you monitor the same group (subjects) over time. Yes?
Assuming I am correct, then you want to use repeated measures in survival analysis.
The methods and platforms in JMP were developed for reliability analysis, but it is the same thing. Just be warned that the words and labels are for reliability engineers and not biologists, if you know what I mean.
Set up your data to look like this example:
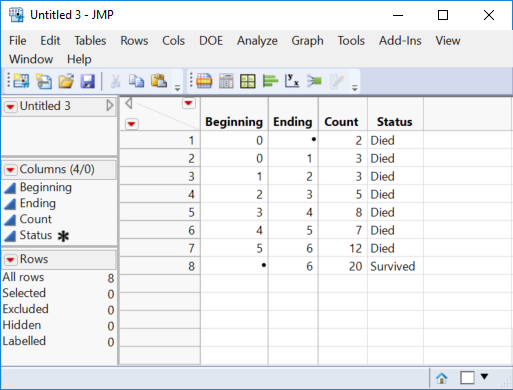
In this example, I have a total of 60 subjects that I monitor from the start of a 6 month program. I need two columns to represent the response (life) because I am not monitoring the subjects continuously but at monthly intervals. This process leads to interval censoring. I don't observe the exact life but instead I observe that it occurs during an interval.
- The first row represents the start of the test. In this simulation, 2 subjects died at the start.
- The second row represents the number of subjects who died between the start and the end of the first month (3).
- The third row represents the number of subjects who died in the second month (3), and so on.
- The last row represents the number of subjects who did not die by the end of the study (20).
I added another column, Status, to indicate the status of the observations but it is not necessary for the analysis. Perform the analyis by following these steps:
- Select Analyze > Reliability and Survival > Life Distribution.
- Select Beginning and Ending and click Y, Time to Event.
- Select Count and click Freq.
The launch dialog should look like this:
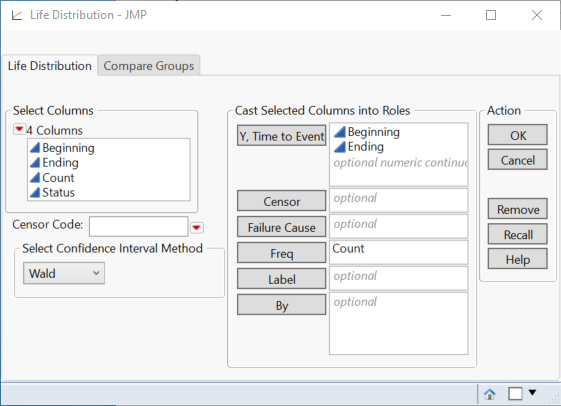
(Note that the Censor analysis role is for right censored data. It is not needed when two response columns are used for interval censoring.)
Click OK.
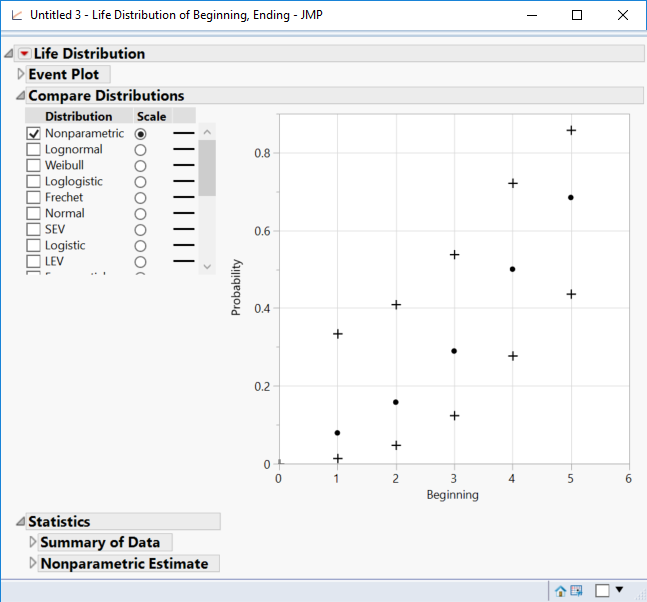
The initial analysis is based on the non-parametric estimates of the failure probability from the Kaplan-Meier product limit. (You can click the red triangle at the top to change it from a Failure plot to a Survival plot.) You use the check boxes and radio buttons on the left side to scale the plot and fit a distribution model, respectively, to the data. Let's fit a normal distribution. Check the Normal check box and click the Normal radio button:
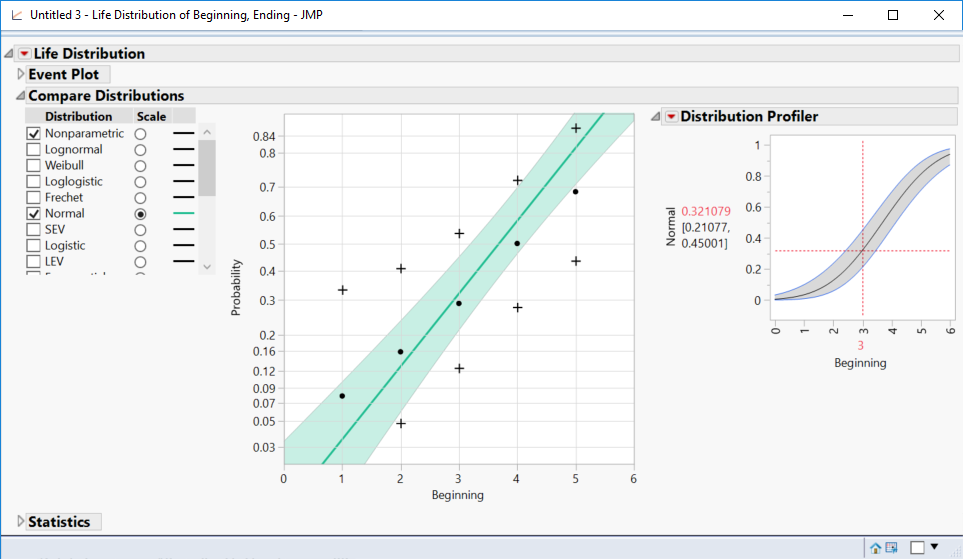
After selecting a model, use the Statistics outline to obtain the desired results. For example, your LD50 is available here as the quantile corresponding to a failure probability = 0.5, so estimate LD50 with the Quantile Profiler:
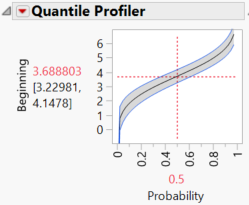
The LD50 in this example is 3.688803 months (3.22981 months to 4.1478 months with 95% confidence).