It's a vector in the mathematical sense -- that there is a list of values rather than a single element.
I'm not sure how else to describe a set of 5 indicator values.
In each observation, an assessor is indicating for each of 5 questions, whether it was true or false (on or off, 1 or 0). It's a basic binary indicator. What is not understandable about a binary indicator response???
The values are judgements by people who are assessing each of 5 possible 'reasons' in each observation. Each of the five responses can be yes or no, without exclusion of the others being yes or no. So, theoretically, the response vector could take on 32 different combinations.
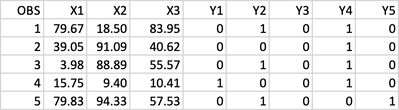