Hi @frankderuyck,
I'm not sure to have understood the link between your covariate factors and the mixture factors.
- Are the 6 covariate factors linked to one batch type (row 1: properties 1, 2, ... 6 correspond to first batch) ?
- Are the batches related to one mixture factor, or several ones ?
What I would like to understand is the link between batches and mixture(s) factor(s) (to better understand the assumed model).
If you have mixture factors A, B, C, ... D, are the batch related only to one factor (A1, A2, A3, ...), or to several factors (A1, A2, B1, B2, B3, C1, C2, C3, ...) ?
- Would you like all batches to be tested ? Or perhaps based on the covariate table for the 20 batches, a representative subset (based on covariates values) could be identified and used in the design generation?
Concerning the model, you may expect some redundancies when specifying terms depending on the assumed model. For example, as sum of mixture factors is equal to 1, main effects for non-mixture factors and interactions between non-mixture factors and mixture factors can't be present independently in the design as they are aliased (but JMP will inform you and deleted these terms if you have these redundancies):
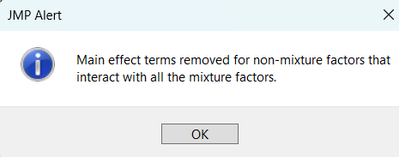
What is your experimental budget ?
If you can provide more infos/screenshots about your earlier tests, it could help frame the topic and better help you.
Initiating the discussion,
Victor GUILLER
"It is not unusual for a well-designed experiment to analyze itself" (Box, Hunter and Hunter)