I wanted to know whether due to climate change, the precipitation in the last 16 years (2004 to 2019) had changed from the previous 15 years (1989 to 2003). Our hypothesis is that the weather patterns has changed and precipitation in the last 16 years had increased.
Here is the annual average data for different years.
Year
|
Precipitation (mm)
|
1989
|
384
|
1990
|
446
|
1991
|
471
|
1992
|
491
|
1993
|
507
|
1994
|
494
|
1995
|
356
|
1996
|
515
|
1997
|
438
|
1998
|
651
|
1999
|
487
|
2000
|
358
|
2001
|
353
|
2002
|
365
|
2003
|
396
|
2004
|
427
|
2005
|
551
|
2006
|
390
|
2007
|
298
|
2008
|
554
|
2009
|
451
|
2010
|
428
|
2011
|
571
|
2012
|
448
|
2013
|
818
|
2014
|
514
|
2015
|
654
|
2016
|
498
|
2017
|
429
|
2018
|
411
|
2019
|
529
|
I run this data using JMP after representing previous 15 years by X and last 16 years by Y. see below.
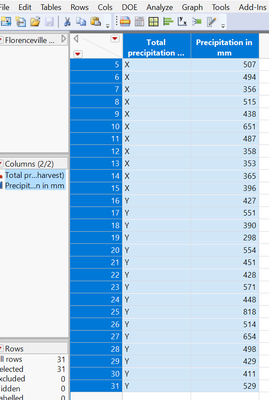
I performed permutation test. See below:
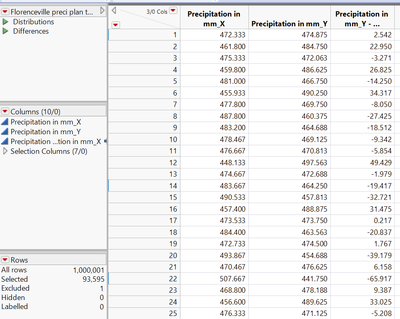
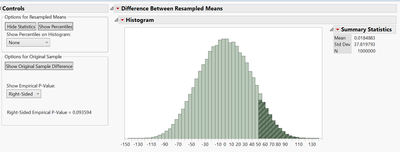
Results show that 91 per cent time I could say that the precipitation increased in the last 16 years (2004 to 2020) than previous 15 years (1989 to 2003).
My questions are as follows:
- Is the analysis correct?
- Should permutation performed with or without replacement?
- Is there any other method, which can give us better results for this kind of analysis for example “the linear trend model?”
Other questions:
When a frequency data ranges from 0 to 30 and there are so many zeros in data, should the data be transformed? Like taking the square root of each number, taking logarithm of each number (+.5) before fitting a “regression test” or “permutation test”?