(I needed to extend the question, the update is below the images)
Hey everyone,
I am looking for some advice. I have performed a definitive screening design (JMP 11) with 3 factors, 1 response (9 experiments) three times. I augmented the design and entered the new data. The problem is, that some combinations produce very varying results. I have repeated some of these experiments additional three times and the variation seems to be "inherent".
My question is how to treat these replicate runs. When I augment the design to have 2 replicate runs (3 runs in total), my regression looks pretty bad.
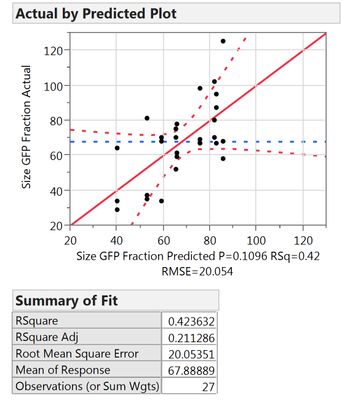
However, when I average my trial runs first and then perform the regression, I get much better results.
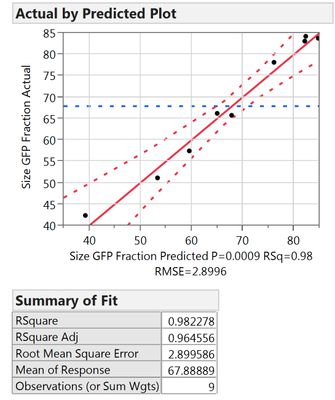
But when I do the averaging first, I think I disregard the information about the standard deviation/variance, which I would like to include (and maybe penalize, because we would like to work in a "stable" region).
Is there any way to include this into the DoE? Maybe use the SD as a response and minimize it?
Edit:
I've thought about my problem and would like to extend it a "little" bit. I thought that I could use the SD as weight for my data, in order to take into account that some conditions used for the experiments lead to very varying results and others are more reproducable. So I read about weighted least square regression and now I am very confused because choosing the weight appears to be non-trivial. Thus my question extends to: If I use a weight, which one would be appropriate. Currently I have thought about and tried the following approaches:
- Use the SD (from what I have seen, it should used as 1/SD or 1/SD^2). This sounds very reasonable to me but on the other hand I have read that one needs lots of replicates to have a proper estimate of the SD (in the range of dozens), otherwise this method is not accurate enough.
- Use one of my responses as a weight. I am also measuring the so called polydispersity index, which gives me some information about the homogenity/heterogenity of my samples. The results looks quite good, but I am not sure if it is allowed to use a response a weight (I think I would introduce some kind of bias?)
- Use the residuals. I have found this in a lecture handout. The idea is to make a non-weighted fit first and the use 1/(residuals)^2 as the weight. But againg, I am worried to "push" the resulting fit into a wrong direction.
Thanks a lot!
Nachricht geändert durch Roland Goers