Hello Community,
I have a question about a case of finding out whether there is any important correlation between an ordinal response variable and a continuous predictive variable.
This is my data (I also attach the JMP file just in case):
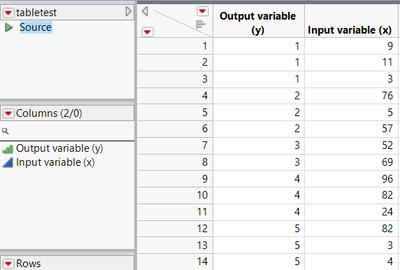
At first I thought of using “Fit model platform” and then displaying Spearman’s coefficient, but then I found this information in a JMP book:
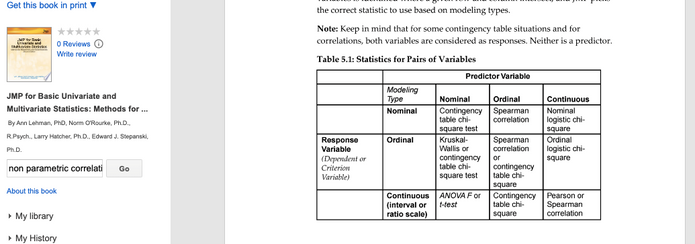
According to this table, when dealing with an ordinal response variable and a continuous predictive variable, I should work with ordinal logistic. I conducted this in the “Fit model” platform and it gave me these results (script present in the file attached):
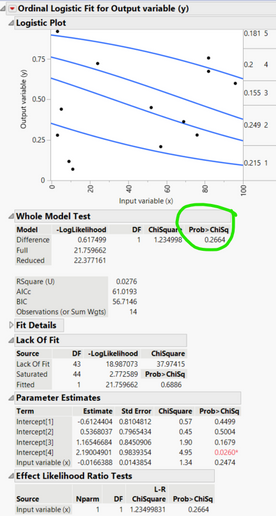
I had to go through several sources of JMP documentation and videos to try to understand these results. I found a lot of sources, but most of the time they focused on nominal logistic cases and were too focused on describing each part of the report rather than on practical meaning and most useful information to get from this. My objective is not to have an in-depth understanding of the math/stats, but rather be very practical on what these results are telling me.
What I could understand (please correct me if I’m wrong) is that the value with the green circle in the figure above tells me that my model is not very good. For my case, using a single input variable, could I use this result to finally say that there is not any sort of correlation or association between my input and output variables ? > this is the main question I want to answer.
I was also interested in understanding the results of the prediction profiler:
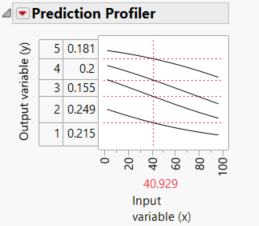
Are the proportions in the Y axis telling me the percentage of probability of having a value of 1, 2, 3, 4 or 5?
Finally, too short questions that are somewhat related to this topic:
a) In the case of having a Likert scale (intensity scale) for my output variable, I know that it is mostly an ordinal variable and therefore to be treated with ordinal logistic too. However, If I have several measurements for each single trial (row) and I average the values, now the result begins to look like a continuous number. Could in this case treat those means as a continuous variable (instead of ordinal)?
b) If my output variable are frequency values. Is it better to treat it as ordinal variable or could I treat it as a continuous variable.
Thanks for reading,
Julian