If you happened to catch my previous blog post, you will know that I am a big proponent of the use of covariates in the Custom Designer when the need arises. For JMP 16, we have made some improvements to make it easier to use covariates.
New Look
The first thing you may notice is a new place for covariate specification. Under the Factors outline, there is a special outline labeled “Covariate/Candidate Runs.” The hope is that by bringing it more out into the open, more users will become aware of the ability to use covariates.
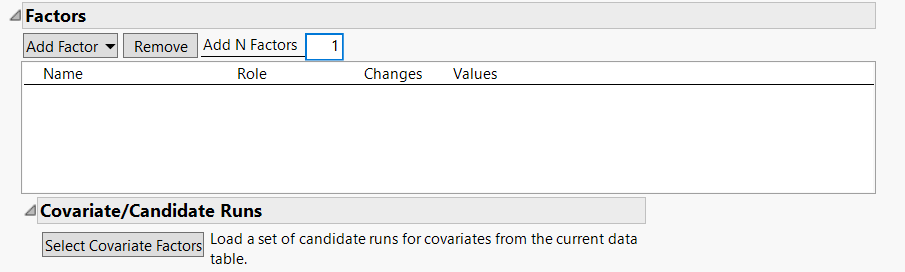
We still need a data table to load covariates, so we will use the “Runners Covariates.jmp” data table from sample data here. This table has a list of 100 runners who are candidates for an experiment on running shoe wear (first 10 shown here):
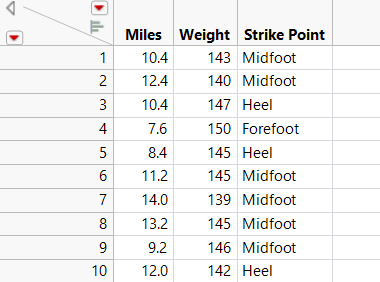
Say we wanted to use a subset of 24 of these runners with an additional controllable factor for shoe type (A/B). Clicking the Select Covariate Factors button, we can choose all three factors as covariates:
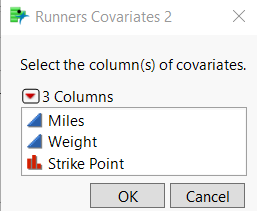
We now get an embedded table within the Custom Designer:
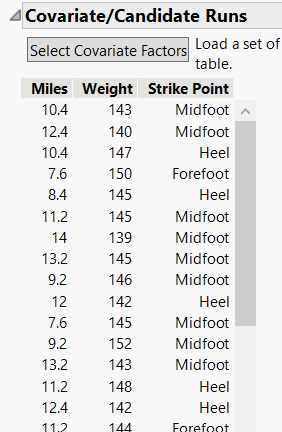
We can now add a factor for Shoe Type:
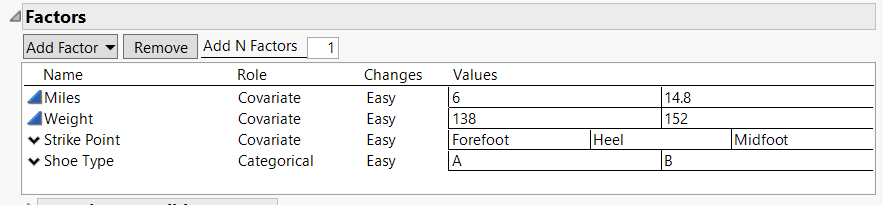
and modify the model, however we see fit.
Additional options
Note that the rows of the table with the candidate runs for the covariates in the Custom Designer are selectable. The reason for this is that there are situations where we want to ensure certain runs are in the final design. For instance, materials may have been used in the past or the experimenter has a commitment to use some certain candidate runs. Another possible case is to use a candidate set by selecting the “Include all selected covariate rows in the design” so the designer will make sure to all the selected runs in creating the design. This is the look of the design generation outline with covariates:
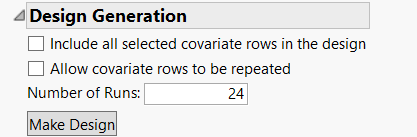
Another possibility is to use a candidate set for adding additional runs to an existing design, effectively an Augment Design using covariates. In this Discovery Summit Europe talk, one of the ways to create a design was finding a subset of the full factorial where the predicted value at a given point was above a specified value. The next batch of 48 runs was chosen from this set while accounting for the points in previous iterations of the design.
The “Allow covariate rows to be repeated” allows the Custom Designer to choose any of the rows more than once, which is useful when you just want to supply runs in a certain form. While you can have the same effect by replicating the design several times, it is not always clear how many replications of a design are sufficient.
One more thing
Much of the work for handling covariates is going on behind the scenes. Under the covers, previous versions of JMP only focused on D-optimality for the covariates. In JMP 16, the covariate rows are chosen according to the specified optimality criteria. The integration between covariates and controllable factors is also better handled to search through more possibilities.
Thanks for reading, and I hope you find the ability to use covariates handy in your own experiments!