We were delighted to feature the amazing father-daughter duo, Dr. Chris Nachtsheim and Dr. Abby Nachtsheim on our first Statistically Speaking of 2023. We have enjoyed past events with Chris like this excellent plenary talk at a past Discovery Summit Americas (well worth seeing again!). And we were super-interested to learn more about Abby’s fascinating projects.
Chris is the Frank A. Donaldson Chair of Operations Management in the Supply Chain and Operations Department of the Carlson School of Management at the University of Minnesota. His research interests span optimal designs of industrial experiments, predictive modeling, and quality management. He has co-authored several books and has numerous articles published in the statistics literature. He is a Fellow of the American Statistical Association and is also the recipient of many awards, including these highlights:
- Four-time recipient of the American Society for Quality Brumbaugh Award
- Two-time recipient of the Lloyd S. Nelson Award
- Three-time recipient of the Jack Youden prize for best expository paper in Technometrics.
Abby is a statistician in the statistical sciences group at Los Alamos National Lab. She collaborates regularly with physicists, chemists, biologists, computer scientists, and engineers to develop solutions to complex, interdisciplinary problems. Her research interests center on the design and analysis of experiments, and she provides DOE expertise to a broad range of applications at Los Alamos National Lab. She is also an active member of the national statistics community, and currently serves as President of the Twin Cities chapter of the American Statistical Association.
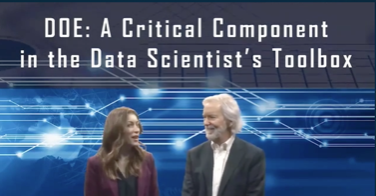
Their Statistically Speaking plenary was so engaging as evidenced by the many Zoom reactions and comments in the chat, like these:
- “I *love* DOE! It has literally changed the effectiveness that my R&D Scientists worked with.”
- “Love the collaboration of Abby’s work!!!”
- “Enlightening presentation, thank you!”
- “This is amazing.”
- “It's a shame DOE is not used more in Data Science, as it can be very helpful to create the most informative and representative training dataset possible.”
- “Thank you for an amazing discussion.”
Their plenary also inspired so many good questions, several of which went unanswered during the limited time we had for Q&A, but Abby and Chris have kindly agreed to answer more of the great questions asked by viewers in this blog post.
For these companies that have departments of experimentation or causal modeling, what other skills might they need, especially when an experiment may not be feasible?
You need to have a good understanding of regression and predictive modeling, probably also the ability to code in R and/or Python, and perhaps familiarity with some causal modeling techniques. And importantly, have a good understanding of the difference between observational and experimental studies. Experiments give cause and effect. Observational studies give hypotheses.
Can you use Plackett-Burman design for chemical formulations to evaluate individual components? Or should mixture designs always be used? I have used Minitab’s screener and modeling tools under the DOE assistant for mixtures but have not used the mixture design as I'm typically evaluating more than three factors for screeners and around 3-4 for modeling.
Yes, you can use a Plackett-Burman design for chemical formulations. If the sum of the component percentages is equal to 100% or some other constant (say, 40% of the total mix), you would need to either, 1) use a mixture experiment, or 2) leave one of the components out and use a more standard design such as Plackett-Burman. Leaving one out is called the slack-variable approach. Mixture designs, of course, do not apply if the sum of the component percentages is not a fixed constant.
What are the advantages/disadvantages of using DOE rather than stochastic gradient descent for hyperparameter tuning? Can these methods be used together (maybe DoE first?) Neural Networks? Could they be considered? Could they be considered for the complex case?
Stochastic gradient descent is an important optimization technique, often used to fit the (perhaps millions of) parameters in the neural network for a fixed set of hyperparameters. But I wouldn’t use it for hyperparameter optimization---it would be expensive relative to the use of a designed experiment.
What about PCA/PLS? Can they be “assisted” by DOE?
From discussions of the very topic with Professor Dennis Cook at the University of Minnesota, we know that there are theoretical reasons why PLS (Partial Least Squares) can *not* be assisted by DOE. And while it may be possible, we are unaware of any useful connection between DOE and PCA.
Where do you see the biggest opportunities for statisticians working in modern DoE for Big Data to have an impact? I know that A/B testing seems to be emerging as a new area with lots of potential (see Nicholas Larsen, Jonathan Stallrich, Srijan Sengupta, Alex Deng, Ron Kohavi, and Nathaniel Stevens, “Statistical Challenges in Online Controlled Experiments: A Review of A/B Testing Methodology.”
Statisticians in DOE can have a major impact on A/B testing, both through the efficient use of subjects and through the implementation of multifactor experiments that are capable of estimating interactions among factors---that is, going beyond OFAAT testing. But we suspect the greatest impact will come from involvement in the development and testing of AI models.
Are successful companies using experimentation?
Absolutely. The degree may vary, depending on the product, but experimentation is widely used in the best companies. There is a lot of literature on this. One place to start is this recent Harvard Business Review article: SCHÖPPNER, F.; THOMKE, S.; LOVEMAN, G. W. Act Like a Scientist. Harvard Business Review, [s. l.], v. 100, n. 3, p. 120–129, 2022.
In discussions I hear, ML sounds 'preferred' over DOE. How would you describe the benefits of modeling based on DOE's (not ML) and also the approach of using DOE to collect data for ML analysis?
We don’t see ML and DOE as competing methods. DOE is simply an efficient way of collecting data to learn how various controllable factors affect a response of interest. ML is a way of fitting highly complex models to (usually) large amounts of existing data. The data could be observational or could be the result of a designed experiment.
What topics should be included in a DOE course for Data Analytics and Data Science programs? For example, should we concentrate on topics such as A/B testing that are not normally in a standard DOE course?
In addition to A/B testing, DOE topics should include design and analysis of factorial experiments, blocking, analysis of covariance and screening designs. A good understanding of the difference between main effects and interactions is critical.
How do you assess the quality of your DOE?
Before or after the experiment? Before the experiment, there are many standard statistical measures of the goodness of the design, including the power for detecting the effects we want to see, degree of orthogonality between/among factors, expected precision, robustness, degrees of freedom for error, D-efficiency, etc. Non-statistical: Have we controlled all of the factors that can affect the response? Did we randomize? Is the response measurement reliable? After the experiment: does the statistical model predict reliably? Is the experiment replicable?
Would the Max Entropy designs be useful for estimating parameters for mechanistic/deterministic models?
The max entropy designs we discussed would not be useful in that application. Our designs are tailored to discriminating between two or more models, which could be mechanistic/deterministic.
How do you go about finding the relevant factors for a DOE? My experience is that often we run a DOE and realize we have missed some obscure but important factor and then need to rerun the design.
That is a major problem, and we have also had those experiences. As experiment designers, we must rely on the subject-matter experts to identify the important factors in advance. So first, work with really smart people, if you can. If there is a lot of uncertainty, you can screen a large number of potential factors cheaply and reduce the set to the relevant ones. Then go from there.
Do you have a specific suggestion on how to incorporate DOE into data science programs and curricula?
Focus on A/B and A/B/n testing, but also introduce the concepts of factorial experiments, blocking, analysis of covariance and screening designs. A good understanding of the difference between main effects and interactions is critical.
If you have large observational data sets already, do we still need DOE?
Observational studies cannot prove cause and effect, and most observational data sets amount to very poorly designed studies that are often not replicable. If you want to identify cause-and-effect relationships reliably, you need DOE. Observational studies can be useful for identifying potential factors for follow-up experimentation.
One question we asked during the livestream, but didn’t have a ready answer for it was, “Are there participants who are working with and/or actively interested in DOE to hospital operations improvement? A helpful resource to start might be: Design of experiments in the service industry: a critical literature review and future research dire.... If others reading this blog post know of more resources applying DOE to hospital operations, we invite you to share in the comments. Thanks!
Many thanks to Chris and Abby for taking the time to share more of their statistical expertise. We hope you will watch the on-demand episode of Statistically Speaking to see some truly inspiring applications of designed experiments benefitting us all.