Hi @Jake_b ,
I won't comment here on which platform is best to use or not, @Byron_JMP and @Mark_Bailey are experts in JMP, so I'd follow what they are talking about. I wouldn't be too surprised if each platform had a slightly different algorithm behind when it comes to fitting models, and hence I wouldn't be too stuck on whether or not they match up. For example, in theory you should be able to get a the same answer in both the boosted tree and bootstrap forest platforms if you set all the parameters appropriately. However, the underlying algorithms are different, so the actual prediction formulas will be a little different.
Out of curiosity, do you actually have a theoretical model/formula that you can use in the Nonlinear Platform, or are you using something else for the X, Predictor Formula?
That being said, you should still be able to get the prediction CIs from the Fit Curve platform, albeit with some extra steps. For example, if you take the Big Class.jmp file and fit :weight by :height in the Fit Curve platform, you can save the prediction formula to the data table. Then, if you do a Fit Y by X (Y= actual, and X=predicted), you can use the drop-down hot button next to "Linear Fit" and save the "Indiv Confidence Limit Formula", which saves the +/-95% confidence interval of the fit. You can the go Graph>Legacy>Overlay Plot, then select the actual, predicted and +/-95% CIs, and you can get a figure like the one below. By the way, I fit the :weight & :height with a logistic 4P model from the Fit Curve platform.
So, if it makes more sense to use the Fit Curve platform, this is at least a way to go about it so you can visualize the results.
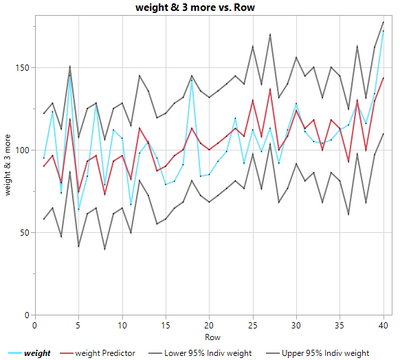
Just something to think about at least.
DS