What you did is not what I suggested. What you have done in the last using "Life Distribution - Compare Groups" is a third analysis in this thread of discussion. What you have done is technically identical to what you have done in your second analysis. That is why you get "same" results. I don't understand the objective, so I will stop trying to guide on your specific data. In the following, I will use JMP sample data sets to make my points, be useful in general to the audience who read this thread.
First, "Competing Risk Mixture" and "Competing Cause" are two different analyses. The terms "competing risk" and "competing cause" may be used interchangeably in the literature. They both take rows of observations, and each observation is a time-to-event observation. And each observation is associated with a cause. But in some experiments, you known the cause; in other experiments, you don't. When you don't have failure causes, you use Competing Risk Mixture. When you have failure causes, you use "Competing Cause". Competing Risk Mixture helps you to guess distributions of individual failure causes, but won't be able to tell the causes of individual observations for sure. Competing Cause fits distributions of individual failure causes, and failure cause is known information. Under normal use cases, there should not be confusion about which analysis to use, and one should not modify data in order to switch analysis types. "Appliance.jmp" is a sample data under Reliability sample data folder to illustrate Competing Cause. There is a Cause Code column in the table. The column "Y2" in "Mixture Demo.jmp", a sample data under Reliability sample data folder, is created to illustrate Competing Risk Mixture. There is no cause information in the table.
Second, when using Competing Cause, there is no need for user to modify data. The software modifies the data for the user to fit the model. Here is what the software does conceptually. I use "Appliance.jmp" as an example.
- For every cause code, create a copy of the entire data. Suppose the current cause code is 9. And I have a copy of entire data of 36 rows.
- Create two new columns: Left and Right.
- For every row, if the cause code is 9, simple copy over Time Cycles to both Left and Right. Row example, the second row.
- If cause code is not 9, copy Time Cycles to Left, but fill Right with a missing value. You may recognize this represents a right censored observation. Take the first row as an example, whose failure cause is 1. Because it is competing cause, cause 1 happened first. If we pretend 1 did not happen, and the system will fail due to cause 9, the failure time should be some where after 11 cycles, but we don't know when. So for this row, the software copies 11 to Left, and fill Right with a missing value.
- Now Left and Right are the new columns that should go to Y Time to Event role, if you want to fit a distribution to cause code 1.
This is what the new data look like:
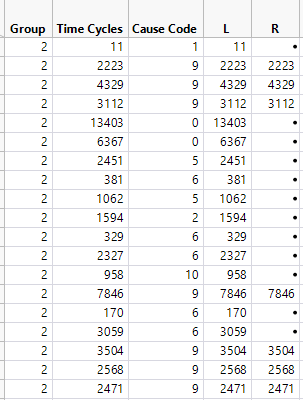
If you analyze the modified data using Life Distribution like this:
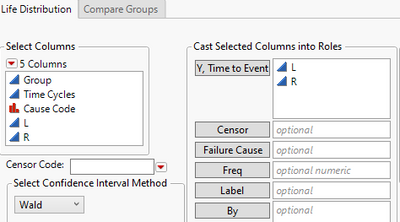
You can get the same Weibull estimates same as those from Competing Cause analysis.
This is the result from Competing Cause by running the embedded script in Appliance.jmp.
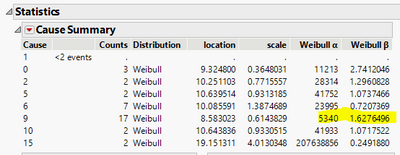
And this is the result from Life Distribution using the modified data.
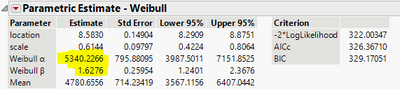
Repeat for all cause code values. And you get the full results of distributions for all causes. The software does all those for the user, and eventually aggregates all fitted distributions into one that is the failure distribution of the system.
To conclude, I want to emphasize, "Competing Risk Mixture" and "Competing Cause" are two different analyses. Decision of using which should be made upon whether there is information of failure causes.
In the end, to your last question, Fit Life by X does not support Competing Risk Mixture type distributions.