Hi all,
I'd appreciate any advice. I'm helping a scientist at my company design a mixture experiment with 5 ingredients. We wish to measure main effects and 1st order interactions.
I asked the scientist to provide mins and maxes for the ingredient concentrations, the test precision standard deviation, and to estimate the effect size of the dominant ingredient. I'm relying on Power to decide how many runs are needed. The effect size of the dominant ingredient is about 20 standard deviations. I find for 30 runs, the power for this ingredient is only 0.168. For 60 runs, it's 0.33.
An effect size of 20 standard deviations is obviously very large, so I don't understand why the Power is so low for this case.
I investigated further by simulating results for 5 instances of the response variable with the appropriate coefficient and standard deviation, and the ingredient with the large effect size is statistically significant (p < 0.001) in all 5 cases.
What can I do? I'm not sure I trust the power calculation for this case. Am I hitting up against a limitation of the power calculation? How can I provide guidance on the number of runs required?
The scientist's goals for the design are perfectly reasonable, and I feel frustrated that I can't give him the guidance that he needs.
Many thanks! I attached a screenshot of the power, the .jmp design, and the text output of the models with the simulated responses, if you are inclined to take a look.
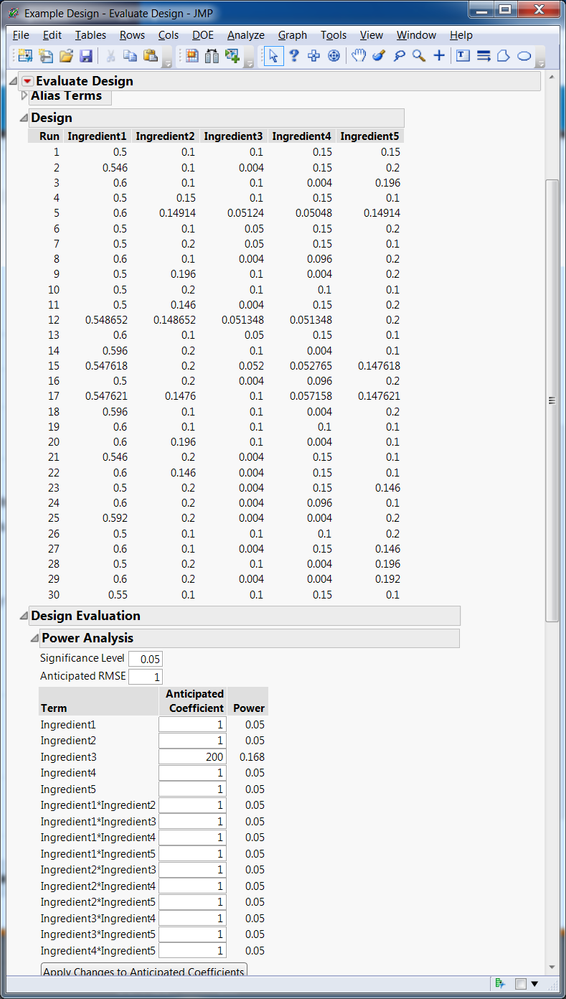