A few more points to add to all of Mark's great advice. Low power in a formulation is typically caused by a narrow factor range, which is true in this case. Further, the concept of power in a mixture experiment is difficult to think through. Power is the probability of detecting a real effect. If X3 has an effect, doesn't it make sense that the other factors (at least in combination) have an effect since they must change in relation to X3? People often think of the power as if the factors are independent when they are not.
Finally, remember that the two-way terms are quadratic terms in the Scheffe mixture model and describe curvature along the edge of your experimentation window. Along that edge, in an unconstrained case, you may have a shape that looks like this:
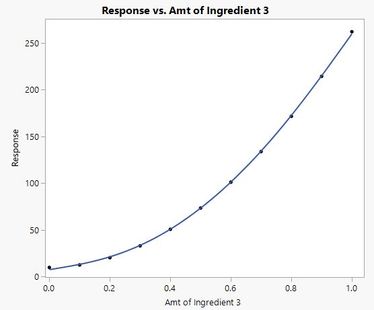
This is indeed a large effect. However, because you are restricting the range to (roughly) 0 to 0.1, you are really estimating this shape:
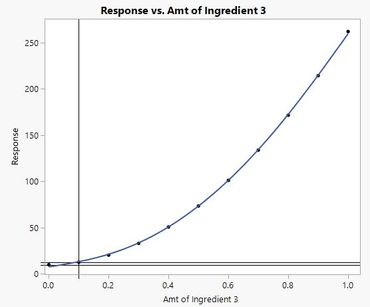
which does not look much better, even when zoomed in:
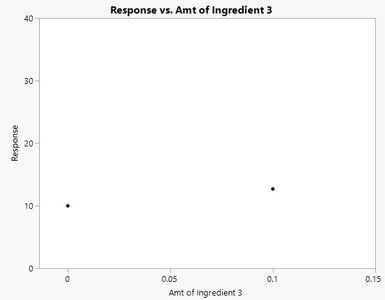
What looks like a large effect, may not be as large as you think due to the interplay with other factors and the restricted range. Put another way, the model would have a difficult time distinguishing between a pure linear model or a pure quadratic model -- they are indistinguishable over this narrow range. Replication alone is not going to adequately fix this kind of issue.
So, power is a good thing, but in many mixture experiments the focus is more on predicting the response. As Mark said, you can get very good predictive models even with low power.
Dan Obermiller