My responses:
"By "causal structure", I assume you mean the identification of assignable or special causes."
Not exactly (realize there are 2 models; The Shewhart model separates the variation into assignable and random and the Deming model separates the variation into Special and Common cause. While synonymous, they are not identical), I mean understanding the relationships between input variables (X's) and response variables (Y's) symbolically represented by Y=f(X). The process of understanding causal structure of starts with hypotheses (these are likely from the engineers and scientists familiar with the process/product). These are hypotheses not facts and are often developed as a result of questioning the process, observation, data mining, etc. There should be a multitude of hypotheses. Once you have a set of hypotheses, you then get data to provide insight into those hypotheses. How you get the data is an important decision. If you are low on the knowledge continuum, the hypotheses might be fairly general. This usually suggests sampling (components of variation studies) to get clues as to where to focus your efforts. This is the intent of SPC. Based on the little information provided, your process appears to be a batch process. Some questions I would have include; How capable and consistent is the measurement component? Is it adequate to discriminate sources of variation both within and between batch? How much variation is there within batch? Is the within batch variation consistent? Is there any systematic variation within batch (e.g., stratification)? How much variation is there between batch? Is that variation consistent? How do these sources of variation compare to each other (e.g., what is the rank order of these components)?
While Moving range charts can provide insight into consistency, they do very little to separate and assign the different components of variation. After all, over time, all of the x's change within subgroup in a moving range chart. If on the other hand your sampling consists of multiple batches, multiple samples within batch and multiple measures of the same sample, you have three layers of components that can be separated. Here is an example of a nested sampling tree:
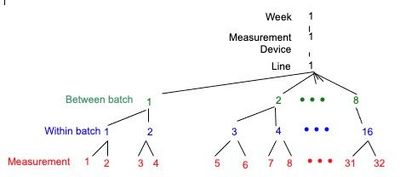
I suggest using hypotheses to determine how many layers you want in your tree (e.g., how many components do you want to separate). KISS, that is Keep It Simple and Sequential. The purpose of the first sampling plan is to help develop a better, more specific sampling plan directed by the data (like perhaps an experiment).
I suggest you read the following to get a better idea of the methodology:
Wheeler, Donald, and Chambers, David (1992) “Understanding Statistical Process Control” SPC Press (ISBN 0-945320-13-2)
Wheeler, Donald (2015) “Rational Sampling”, Quality Digest
Wheeler, Donald (2015) “Rational Subgrouping”, Quality Digest
Shewhart, Walter A. (1931) “Economic Control of Quality of Manufactured Product”, D. Van Nostrand Co., NY
"All models are wrong, some are useful" G.E.P. Box