A lack of fit test compares the variance from the "model not fitting" to the variance of the replicated points (pure error). A significant result means that the "model not fitting" variance is larger than the pure error. The most likely cause is that the model form you are using is not appropriate for the data.
Consider this picture:
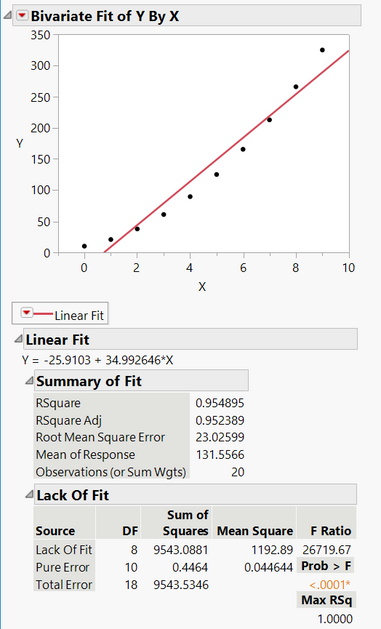
A high RSquare value, yet a significant lack of fit because a straight line does not describe the relationship. Remember that a high RSquare by itself does not mean you have a good model. A high RSquare means you have explained much of the variance in the response, but you still may not have a good model. You must consider other items such as model significance, residual analysis, etc. to determine if you have a good model.
So I would recommend plotting your model residuals to see if there are any patterns. A pattern would indicate what might be wrong with your current model form. Also check to ensure that the residuals follow a normal distribution as that could also lead to a lack of fit situation.
Finally, it is possible to get a significant lack of fit test if your replicated points have an extremely low variance rather than the model form being incorrect. This does not happen that often, but I have seen that occur. In such situations, you should look into determining why or how you achieved such a low variance. That can provide some good insights.
Dan Obermiller