Bias is generally understood to mean a fixed effect on the response, like non-linearity. Your effects, such as repeated calibrations, are random effects. Sometimes in assay development there is a distinction between accuracy (lack of bias) and precision (cumulative random effects). Other situations consider accuracy to be the combination of the bias and the random effects.
The easiest way to design such a study with JMP is DOE > Classical > Full Factorial Design. Enter all your factors as categorical.
Are your 20 samples the same control, different controls, or random patient samples? That is, are you replicating the assay 20 times on the same sample or running different samples? If different samples, is there any replication?
The analysis will require a few extra steps. Select Calibration in the column list and Run in the Effects list and click Nest. Select Calibration and Run in the column list and Sample in the Effects list and click Nest. Select all the terms in the Effects list, click the red triangle next to Attributes, and select Random Effect. Your Fit Model dialog should look like this:
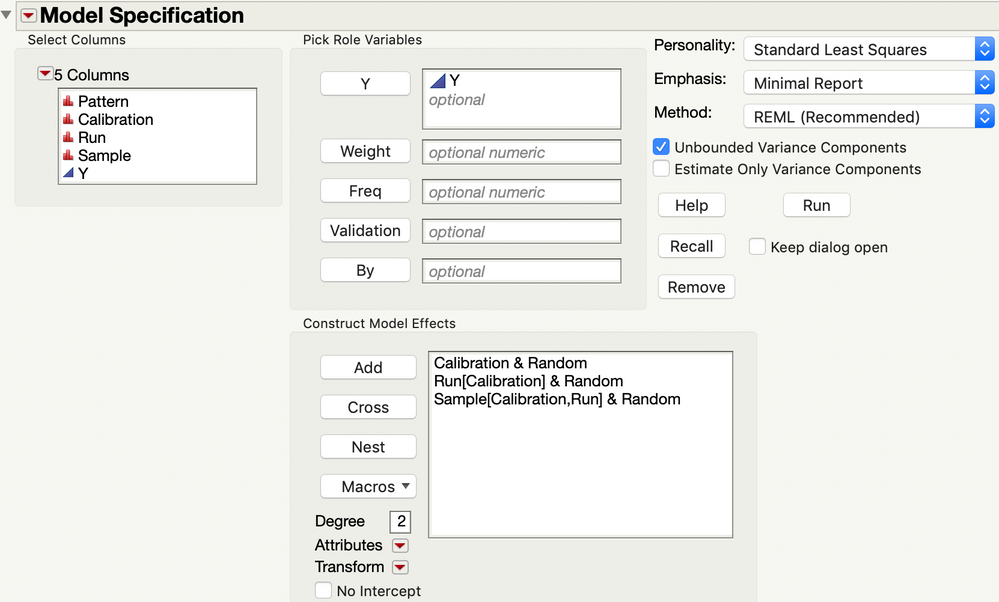
I made a data table as I described above with the updated Model table script and attached it to my reply for your examination.