Hi all,
I'm looking to discuss the best modelling approach in JMP Pro 16 to predict time series concentrations of chemicals in individual samples extrapolated to today's date. Although the time series function in JMP will predict a spread of data illustrating the range at which the time series will fall under, I want to get more quantitative and predict what individual sample concentrations would look like. For instance, if we had 20 samples in 2021, what would their spread look like? Could I then further use these 20 samples to estimate a median/geometric mean of the concentration in 2021?
Or do I take a neural network approach where I predict the concentration of my multivariate chemical data based on date being the x factor? On attempt of this I get negative concentrations at times, which is not physically possible (but the model obviously doesn't know that). How to I constrain the data to predict realistic values based on the previous spread of data?
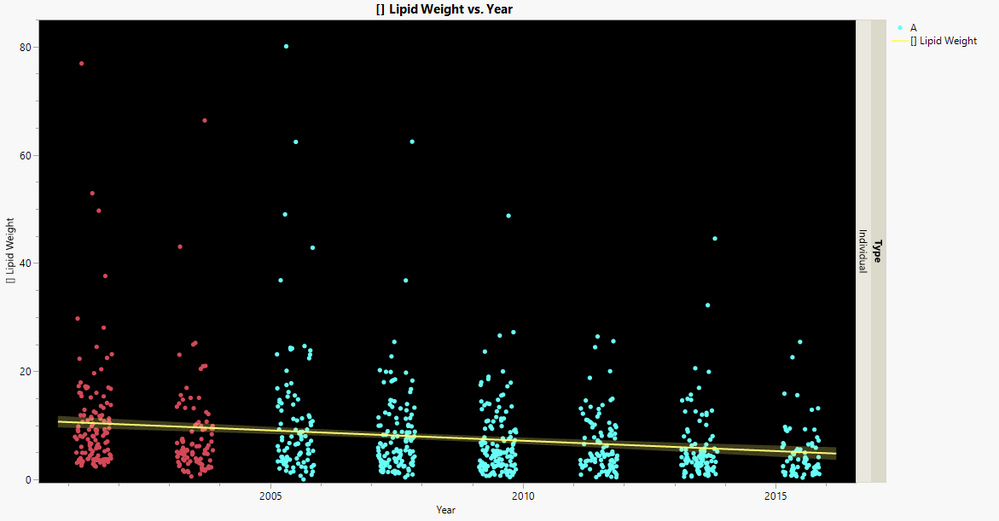
M. Dereviankin