Hi @frankderuyck,
Definitive Screening Design is a "textbook" (classical) design, used for a screening case with high number of factors involved and the possibility to test interaction and quadratic effects, following the basic principles of DoE : effect sparsity, effect hierarchy and effect heredity. I wouldn't categorize this design as an "optimal" design, as the structure is "fixed" (and so are the terms screened by this design).
Concerning your conditions,
- Main effects are completely orthogonal to each other, to 2-factors interactions and to quadratic effects.
- No, there are aliases between quadratic and interaction effects :
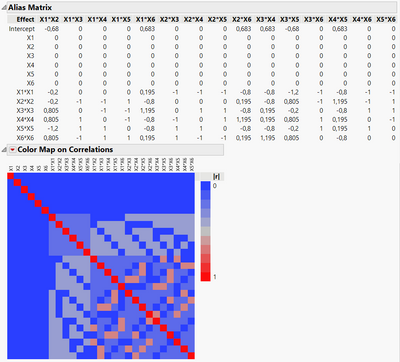
Here is an extract from the presentation of Tom Donelly about the desirable properties of DSDs I attached earlier :
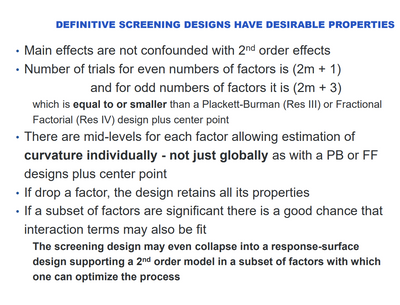
I hope this answer will help you.
You can also search for presentations about DSD from Bradley Jones in the JMP Community, he clearly demonstrates when/where to use DSDs, and the conditions to fulfill (only continuous and few 2-levels categorical factors, high number of factors, no constraint in the experimental space, easy to change factors, ...).
Victor GUILLER
"It is not unusual for a well-designed experiment to analyze itself" (Box, Hunter and Hunter)