Hi @Ahmedhadi,
If you want to compare and select the most "sensible" models, I would recommend compare them with another metric than R² (or R² adjusted), since R² takes only in consideration the proportion of variance explained by the model but not its complexity, so if you add more and more terms, you may end up with an higher R² but you'll have a more complex model that may fit some noise in it.
An information criterion, like AICc or BIC, and/or Maximum Likelihood if you need a different response distribution than the normal one, can be more effective for comparing and selecting models, as they balance the accuracy of the model with its complexity (number of terms included in the model).
You don't need necessarily to try all the possible combination of terms for comparing and selecting the models, as Generalized Regression (JMP Pro) with the different estimation methods and validation criterion is a semi-automated process (each method will stop when reaching the "best" model). More infos here : Generalized Regression Models (jmp.com)
You may also look in the Stepwise platform (in the Fit Model platform, changing the personality to Stepwise), where you can screen "automatically" the possible terms in your model and select the most informative one thanks to a stoping rule/criterion (based on BIC, AICc, p-value threshold, etc... I recommend using information criterion like AICc or BIC). The nice part is that you can follow the different steps involved during the different models creation, and how each step had an impact on the model's quality (in terms of R² and AICc / BIC) :
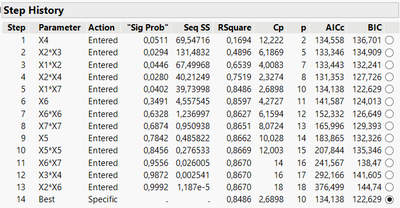
It works quite well, but you may need after all some model fine-tuning, as it can be a greedy approach (it will enter every term that may provide a positive impact for the model).
More infos here : Stepwise Regression Models (jmp.com)
There is also an option to try "All Possible Models", but given the high number of factors you have (and even higher number of possible terms when taking into consideration 2nd order terms), I wouldn't recommend it as it will be too long and too computationally expensive.
I hope this answer will help you,
Victor GUILLER
L'Oréal Data & Analytics
"It is not unusual for a well-designed experiment to analyze itself" (Box, Hunter and Hunter)