Hello:
I have a basic question on R square Adjusted.
I ran an experiment with three continuous factors and measured the response.
Below is the summary of Fit:
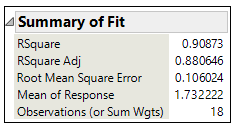
This means that I was able to capture about 88% of variability in my response.
One factor contribution was about 82% and rest was from the other factors and interaction.
Now, I realized that I missed one factor to be included in the study.
My question is:
Q1) Since I have already accounted for 88% variability, does this mean that the factor that I missed can only have a contribution of maximum 12%?
OR
Q2) Adding of this factor in the new analysis can reduce the contribution of one factor from 82% (from previous analysis) to 60% and itself can have another 30%, because if this new factor is significant, this will vary the response as we vary this factor in DOE, this factor was kept constant in the previous run?
I am planning to run a 2^2 full factorial with one replicate and 4 CP (In total 12 runs) just for the two factors (most significant from previous run and the new factor) to practically see its implications but could not resist my curiosity.
Your help is highly appreciated.
Best Regards,
Varun katiyar