Hi,
I think that you might need to add a bit more explanation of what you are trying to achieve. Can you elaborate on "use a set of factors with lots of required level combinations."
Using PCA to reduce the dimensionality of a system like this is a fairly standard approach. The next step is often to build an experiment using the principal components as covariate factors and selecting candidates to give good coverage of the principal components space.
In the attached example with solvents, PCA reduces the solvent properties to 2 components (essentially "polarity" and "bulkiness"). These components are then used as covariate factors in Custom Design to select 9 solvents to estimate the response surface model. You can see the 9 solvents selected cover the PC space, and hence the original descriptor space, very nicely:
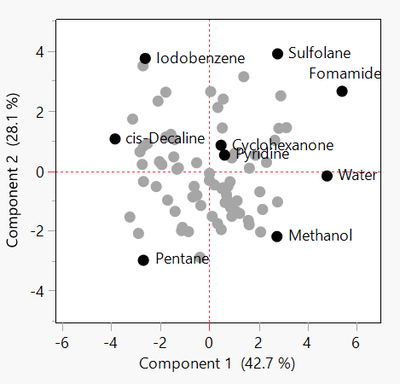
I hope this helps,
Phil