Hi @Archy,
As Mark mentioned, the "Main Effects Plot" from Minitab you've shown here is displaying something quite different from the prediction profiler in JMP *when fitting your model with interactions. If you fit a main effects only model, the JMP Prediction Profiler and "Main Effects Plot" from Minitab display essentially the same thing (update: assuming balanced data, an equal number of observations in each cell of the design -- thanks for the note, Mark).
I've arranged the profiler in rows of two in order to most closely match Minitab for you, Prediction Profiler Red Triangle > Appearance > Arrange in Rows:
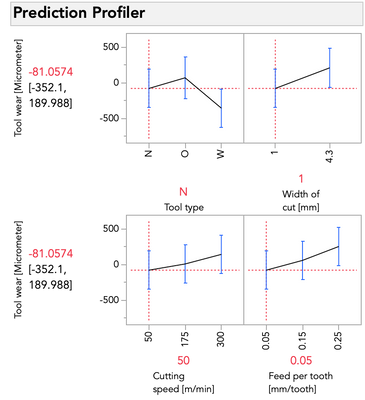
That said, why these plots match in this particular case (a main effects only model with balanced data), but not in the model you fit before, gets right to the meat of what @Mark_Bailey was explaining, and is a critical thing to understand about the choices we make in models.
The prediction profiler in JMP displays the conditional relationship between Y and a given predictor, given the level selected of the other predictors, whereas the main effects plot from Minitab is essentially ignoring the other factors and displaying the average relationship between Y and a given predictor. So what does that mean, and why does the output from JMP and Minitab match in the case above, where I fit a main effects only model in JMP? Let's take the initial model that you first showed (and what is saved to your data table).
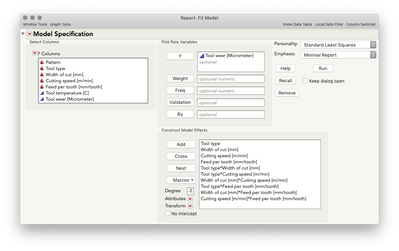
Putting aside for the moment the point Mark made about some of these terms being inestimable (which is certainly important, but is a separate point than this post), this model aims to understand (i.e. statistically estimate) the main effects for each predictor as well as the two-way interactions between each pair of predictors. These interactions are key to the difference between a main-effects plot, and the prediction profiler because the interactions allow our model to capture relationships between Y and each predictor that can be different when the level of another predictor changes. Let's look at the profiler from this model, and I'm going to change the level of Feed per tooth. As I do, look at the relationship between tool wear and tool type at the far left:
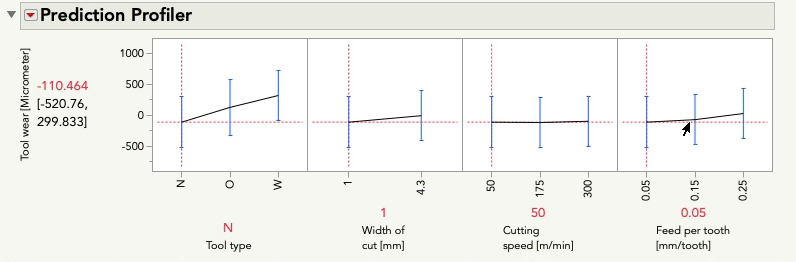
You'll notice the relationship between tool wear (y) and tool type at the far left is different at different levels of feed per tooth. There is an interaction between those variables. There are also interactions among tool type and the other variables. A "main effects only" plot simply averages over those different relationships to arrive at the average, unconditional relationship. That is what minitab shows in the main effects plot, and that is what JMP will show in the prediction profiler if you fit a model with no interactions, like this one:
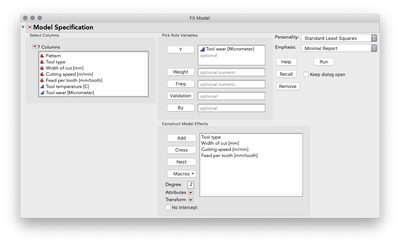
I'll do the same exercise with the profiler below changing feed per tooth. Again, look at the relationship between tool wear and tool type: 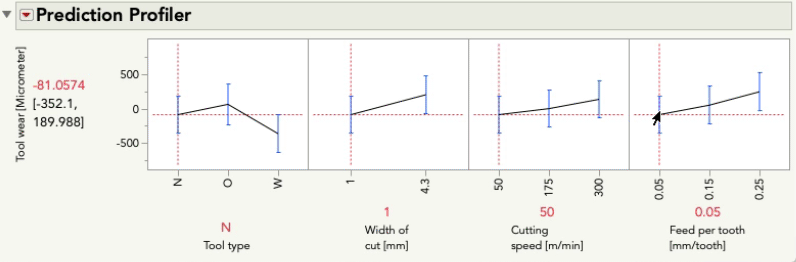
This time you'll notice only the intercept changes -- that is, the relationship between tool wear and tool type is the same pattern, but the prediction for tool wear is only higher or lower by the amount that feed per tooth contributes. These effects are simply additive... there is no possibility for an interaction because we did not give the model the possibility to fit/find one.
I hope this helps clarify the difference you're seeing between the main effects plot in minitab and the prediction profiler in JMP.
@julian