First of all, an interaction effect in the response means that the effect of one factor depends on the level of another factor. In this case, if the slope (represents effect of changing log concentration) is different (not parallel) for the curve type, then the slope depends on the sample and there is an interaction effect. on the other hand, if the slope is the same (parallel) for each type, then there is no interaction effect. Most scientists and engineers mistakenly fit a separate model to each sample type and then try to compare them. This way of working is inferior for several reasons.
Second of all, JMP matches the output as far as it goes. The Regression Plot in Fit Least Squares is the same as the plot shown at the end of the PDF under Graphics. The Means and Standard Deviations report in Oneway platform (Y: Response, X: Level, and By: Type) is the same as the summary tables under Observations. The Results section can also be reproduced but it is not clear how they modeled it. It seems that they performed more partitions of the sum of squares than is usual and I cannot reproduce that level. In particular, I cannot achieve the R square values because they included additional factors. I think that it is the Row and Column effect from the Latin Square design. Also, there is no need to perform an ANOVA and a Regression analysis. That distinction is artificial.
Here is what I get from the ANOVA:
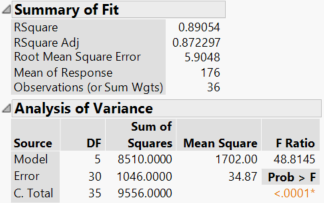
Separately, I get the sum of squares for the individual terms:
I don't have time to add the Row and Column values to the example data table. If they were added, then I could show you how to include them in the analysis if you need the help.