Hi @Ashya,
Welcome to the community!
I agree that the location is probably a good candidate for a random effect, but I would think plant varieties would be fixed along with treatment. It wouldn't necessarily be wrong to treat variety as random, but it seems a bit odd unless the varieties you tested were selected to be a representative sample of all varieties that you would like to generalize the results to. Having other fixed effects other than treatment does not diminish your ability to compare the efficacy of the treatment across plant varieties.
You can fit the appropriate model several ways in the Fit Model platform if you have JMP Pro. The Mixed Model personality in Fit Model is preferable if you have Pro. If you have just have base JMP, you can specify your model this way: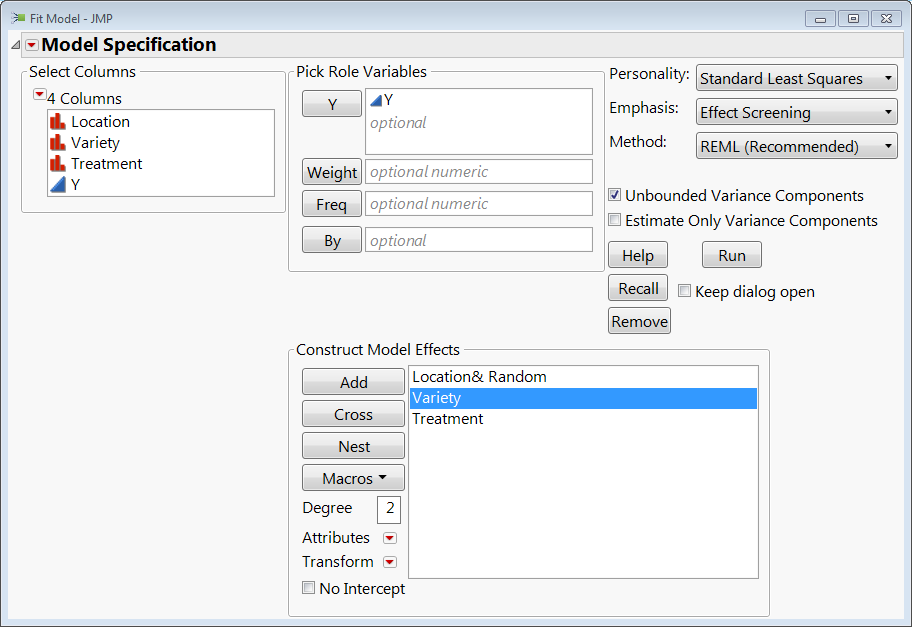
Again, you can treat variety as random if you really want to. I would definitely not exclude it, because if it does explain a lot of the variance, excluding that term will cause all the variance explained by variety to go into the residual noise, and your test for the treatment effect will by less powerful.
As far as checking assumptions, you'll want to do that based on conditional residuals rather than regular ("marginal") residuals because the marginal residuals don't consider random effects and may cause the diagnostic plots to look like they fail the assumption. To get the conditional residuals without JMP Pro, go to the red-arrow in the report window > Save > Save Conditional Residuals. Then you'll need to plot them in distribution to check normality with a normal quantile plot or Shapiro-Wilk test for goodness-of-fit after fitting a normal distribution to the conditional residuals.
You can similarly plot the conditional residuals on a run chart to check for independence. If you want to create a residual vs. predicted plot, you'll also want to save the conditional predicted values and then construct the plot in something like Graph Builder.
If you do have JMP Pro, you can get all the conditional residual plots within the Fit Model report with the Mixed Models personality, which is really nice.
-- Cameron Willden