Hi mkrb,
As Lou suggested, a stacked format of these data will be more amenable to analysis. It seems as though a mixed effects linear model would be appropriate in this situation. If you're using JMP Pro 11 you can use the new mixed model personality in Fit Model, or if you're not using Pro or are using an earlier version of JMP, you can still do the mixed model through standard least squares by marking effects as random. Here are the steps I went through to generate that analysis:
1. To stack the data, I used Tables > Stack. I then added the four response columns to the "Stack Columns" section, and renamed "Data" to "Y" and "Label" to "Condition"
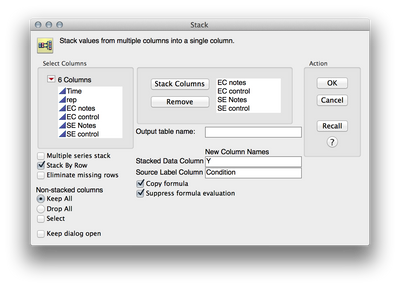
2. We need to separate out the levels of your two factors (Ec vs Se and notes vs controls) if we wish to analyze these factorially. We can accomplish this by twice using Cols > Recode to create two new variables. First select the "Condition" column in the dataset and then select Cols > Recode. Here are the screen shots of the recoding I did, and be sure to select "New Column" rater than "In Place" for the new column (otherwise values will be written over). What these will do is create two new columns that have the levels of each factor independently. This is important when we define the mixed model later.
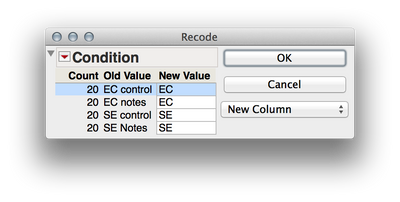
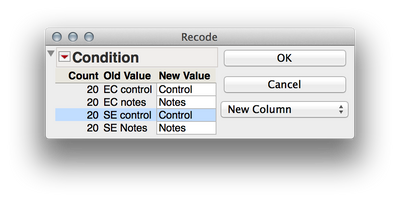
3. Rename the two new columns to have reasonable names. I'm using bacterial strain and surface.
4. We can now define the mixed model, which will take into account (i.e. explicitly model) the statistical dependence among the data points (introduced by taking repeated observations on the same experimental unit). We'll be using Analyze > Fit Model. From this point forward there will be a number of different models you can fit, each with their own assumptions about the covariance structure of the data. I like to start first with a maximal model with random slopes and intercepts for the experimental units across the fixed structure. Simulation studies have shown these models to generalize better (e.g. http://www.sciencedirect.com/science/article/pii/S0749596X12001180) and I find the meaning of the denominator error in each test to be more interpretable (e.g. the degree to which the magnitude of some effect or interaction differs across the experimental units). Here are the general steps to produce such a model when your entire experimental structure is replicated for each experimental unit (which is what you have here - each replicate is measured on all experimental levels). This heuristic is not appropriate when you have units nested inside of other factors.
a. Full factorial (macro) of only your fixed factors (or factorial sorted, which will produce an ordered list)
b. Full factorial (macro) of your fixed factors AND experimental unit (JMP will not duplicate terms, so this produces only the sources for the experimental units and crosses with the experimental units). Ensure that the column identifying the individual units is modeled as Nominal.
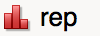
c. Mark all effects associated with your experimental unit as Random Effect
d. Remove terms for which you do not have degrees of freedom to fit*
*This is actually optional. JMP is intelligent enough to know when an effect is confounded with residual error and will remove those terms from the model.
The above is more simple than it looks when written out. Here's a quick video of me defining the model with your data:
5. Click Run and you'll see the results of the analysis. The Fixed Effects section is probably of most interest.
I like to turn on the Profiler from the top Red Triangle menu >> Factor Profiling >> Profiler. I also like to turn on the LSMeans plots for each factor, which can be deployed by expanding the Effect Details section, and then under the Red Triangles for each source you can turn on the plots and request further tests. If you want to turn on all plots at once, hold down the control key before clicking the Red Triangle to turn on a plot. Once you have requested the plot you can let go, and this will tell JMP that you want to broadcast the command. If you're on a mac you will use the command key.
I've attached the restructured dataset with scripts saved for the analysis.
I hope this helps!
Julian