Hi @MikeKim,
Of course there is a way to inform JMP that you have discrete numerical factors, just set up your 4 factors as Discrete Numeric with three levels (-1, 0, 1) :
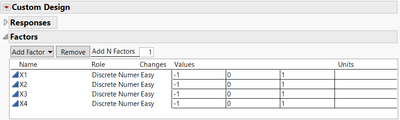
This will add a constraint during the design generation to only use the three possible levels values for each factor in the design :
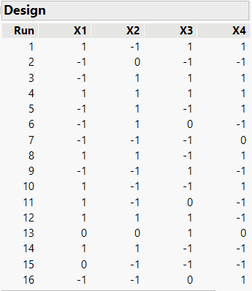
But that doesn't change the fact that design generation for optimal design in JMP is "random-based", please see my previous post about the Coordinate Exchange Algorithm and the response from @Mark_Bailey for explanations and informations. So you might end up with a design having similar performances, but different runs.
The one I generated with the same model (main effects, interactions and quadratic effects), optimality criterion (D) and run size (16) has a similar prediction variance as the D-optimal design from the book (1,86 vs. 1,80 in the book for the specific setting of factors) :
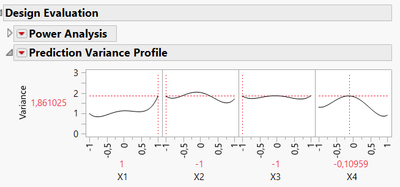
Please find attached the D-Optimal design I created with the discrete numeric factors.
Following the same factor settings and methodology, you should be able to generate a satisfactory I-optimal design, with similar performances and characteristics than the one in the textbook.
I hope this answer will help you,
Victor GUILLER
Scientific Expertise Engineer
L'Oréal - Data & Analytics