*Note: This was my original reply, which at one point disappeared. I responded again before this reply reappeared.
Hi Kynda,
You would need a column to identify the run that produced each set of 4 repeat measures. Make sure that column has a nominal modeling type. In Fit Model, specify your model as usual, but add that column identifying each run and make it a random effect (select the Run effect in Construct Model Effects, and click Attributes > Random).
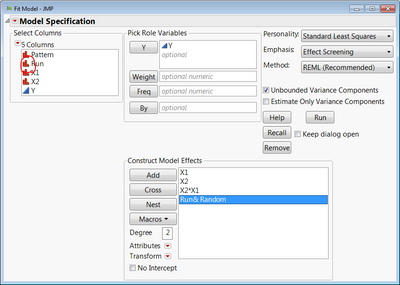
This is all assuming you have replicates in your design (like the stem length experiment has pots 1, 2 & 3 for each combination). If you don't replicate any of those factor level combinations in the experiment, then you're not going to be able to estimate the variance components for the Run blocking factor since it will be confounded with the X1*X2 interaction. JMP will basically ignore the Run random effect and analyze it as if you have 3 replicates (4 of each) rather than repeat measures which would be highly misleading. If you forego the interaction and just model main effects, you will be able to get the variance component for Run, but you will still be contending with how much of that variance component is really just X1*X2 interaction effects.
Here is an example with the stem length experiment from your first reference. I had to nest the Pot random effect into Hours of Daylight and Temperature so that Pot 1 for a given factor level combination is not treated as if it is the same block as another Pot 1 for a different factor level combination.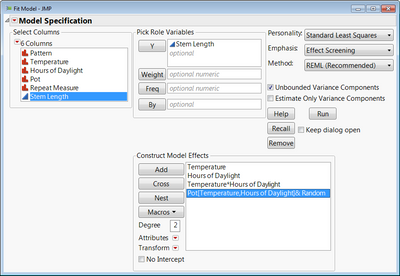
To do the nesting you would, just highlight the Pot effect, and then select Temperature and Hours of Daylight from the list of columns, and click "Nest". I'm attaching a data table with the analysis saved in a script.
-- Cameron Willden