Hi JMP Community,
I routinely analyze binary outcomes such as Response / Non-Response at a single time point in my research using the Nominal Logistic Platform. Now, I need to expand my analysis to incorporate the factor of Time in my model, and I realized that I am unsure about the best approach to do so.
Specifically, in the mock data file attached to this post, I need to evaluate the effect of Time, VAR1, and VAR1 * Time for each level of VAR2 and VAR3 (see plot below), considering possible interactions between VAR1 and VAR2, and VAR1 and VAR3.
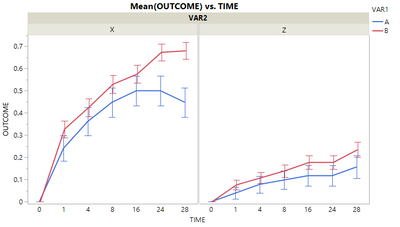
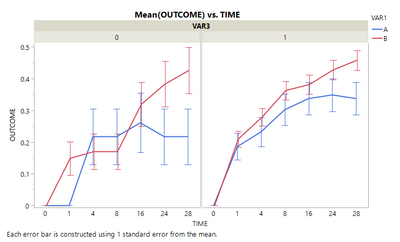
I am familiar with the Repeated Measure Least Square Means model for continuous variables, but I am struggling with the implementation of a similar approach for a Categorical outcome.
JMP 16.1, Windows
All inputs are welcome.
Best,
TS
Thierry R. Sornasse