@garibay90 , not sure about the tests you are running, I haven't read much if anythign about them.
I tried fitting your "X" and "Y" columns of data using the Life Distribution Platform and the the best JMP could come up with there strictly on the basis of Liklihood, AICc and BIC is Lognormal, and Zero Inflated Weibull, respectively. Nether fit is good in my mind: use your eyes to look at the extent to which the points follow and non-linear coherent path on the transformed N-Q plots. If the points don't fall pretty close to the straight line and/or exhibit a curvature, then the transformation is not a very good fit.
Based on my understanding, if you want to run a 2-group comparison using a standard normal parametric statistical hypothesis test (such as ANOVA = t-test for the 2-sample case, then you would need to apply the same transformation to normality for both groups. And the data has to be reasonably normal after you've applied that transformation to both.
JMPs SHASH distribution may be a good fit for your "X" column, actually the best I could find (you can use Analyze Distribution > Fit All and JMP will generate a fit to this one for you). But it won't give you a good fit for your "Y" column. Actually in my experience, you will be very hard pressed to find any transformation which will validly fit this data. You have too many "0.0000" values in your dataset. I've dealt with this issue before and in the end you really have no choice but to run a non-parametric analysis. With data like "Y" I wouldn't trust the inferences I make from the non-parametric tests unless I was reasonably confident that the estimates I am trying to infer on are reasonably representative of the population from which the sample came. For example, if I am running a Wilcoxon test which looks at the score difference between groups, can I rust that the confidence interval of the score mean difference is really representative of the population mean difference? If I choose a test comparing the medians, do I have reason to think that the estimate of the median from my sample in "Y" and the corresponding confidence interval around the estimate, is representative of where the true estimate could be in the population?
I am not aware of a non-parametric test correction for extremely unequal variances. But I could be wrong. For the normal parametric tests, there is a standard correction for unequal variances, but the correction is based on an estimate and is not an exact solution. Look up the “behrens fisher problem” on Google.
If you go with the SHASH distribution to transform your data (which I don't think it works particularly well at all for your “Y” data), then you get evidence of equal variance between groups. You get evidence that there is a statistically significant difference between the means between groups, where the “Y” column mean is 0.62 units lower than the “X” column mean.
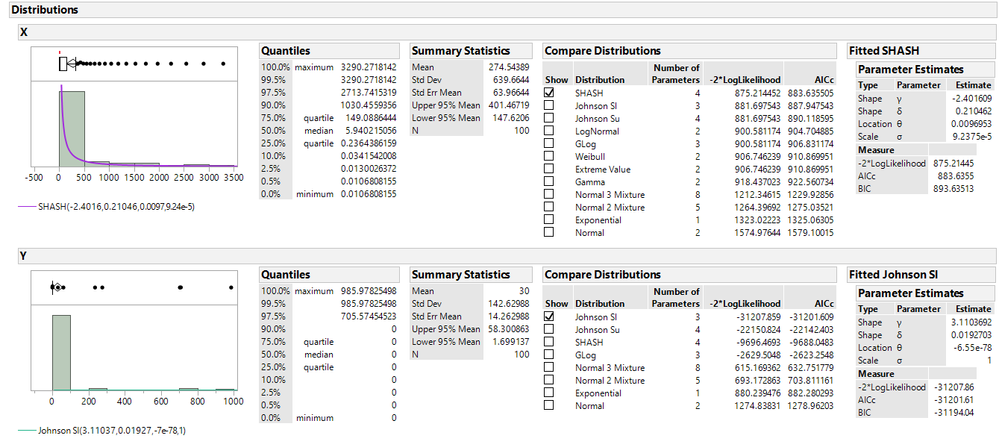
Just taking a step back here and looking at this groups, despite the fact that you have “unruly” data, its pretty clear to me that these groups are behaving numerically differently. You are getting a bunch of 0 values for “Y” but not for your “X” group.
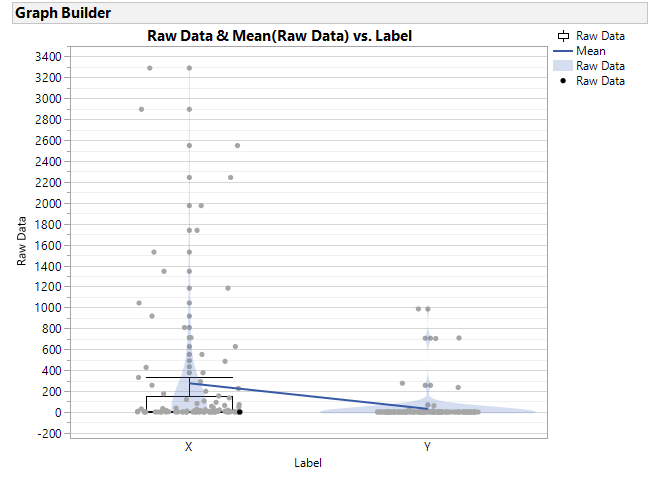
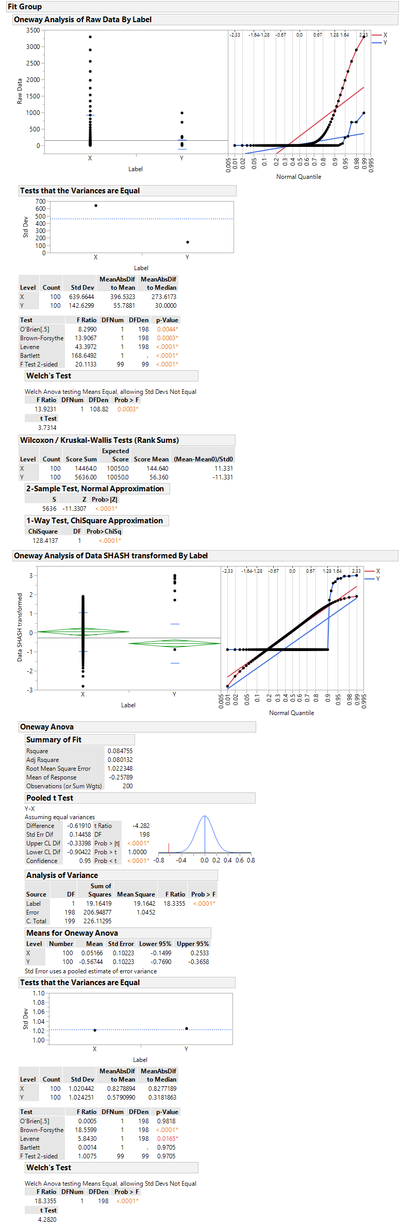