Hello,
I have 3 independent variables, a continuous covariate (Mass), a categorical nominal variable (sex: M and F) and another categorical nominal variable (Site location: North, Middle, and South) and one dependent variable, continuous (mercury concentration). I am interested in testing the hypothesis " If the variation of mass and sex in an organism can be standardized (homogenized, accounted for, smoothed over), then that animal can reflect the bioavailability of mercury in the site of the North location, Middle location, and Southern location."
I tried to explore the use of an ANCOVA, by including my covariate (mass) in my fit model analysis with the categorical variable (sex) and the other categorical variable (site location), as well as the interaction terms. My intention is by accounting for mass and sex within the model fit, I can build an interaction plot for the site locations to see if there is a difference in mercury concentration among them and report their LSmeans as they have been statistically adjusted as if the sexes within those locations had a standardized mass.
Y= mercury concentration
X Mass + Site location + sex + Mass*Sex + Mass*Site location
Interestingly, when this model is ran, this is what my effects test looks like
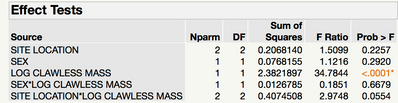
I want to validate that after I have accounted for the covariate as it covaries with sex mercury concentration and site location mercury concentration, we find that there are no statistical differences between the sexes and among the sites.
I am curious if I am treating my independent variables correctly here given the hypothesis I want to test. Any and all assistance will be helpful