The GLM model was fit using MLE. The Generalized Regression model was fit using LASSO. LASSO is a form of penalized regression. It uses L-1 norm for the penalty on the estimates for variable selection. Even if a variable is selected, the estimates are affected by the penalty. The differences that found are due to the penalty. It changes the estimate and the standard error of the estimate so the Wald Chi-square also changes.
But I think that there might be a mistake in the way you set up the model. You need to supply both the successes and the failures for a binomial response. It appears that you only supplied the successes. Also, the number of observations is wrong. You have many more than 4 observations as demonstrated by the N Trials.
I would correct this model as follows. Subtract Successes from N Trials to get Failures. Stack the Successes and Failures columns. Use the column with the counts in the Freq analysis role and the label column (Successes and Failures) as the response or Y role.
The two factors are categorical so make sure that they use the nominal modeling type. The data table looks like this now.
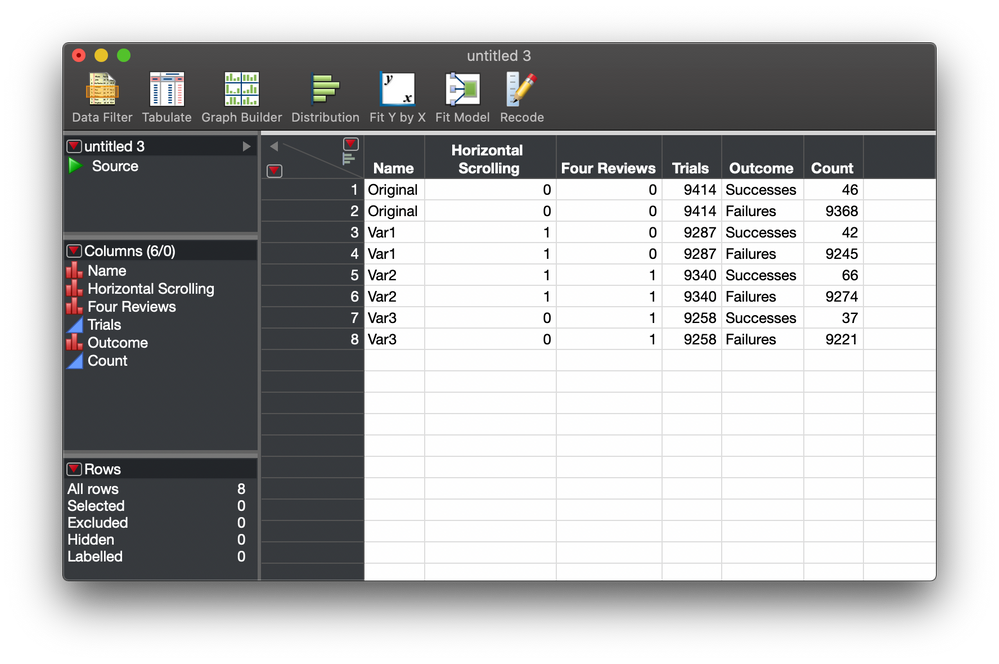
Select Analyze > Fit Model and fill out the launch dialog as follows:
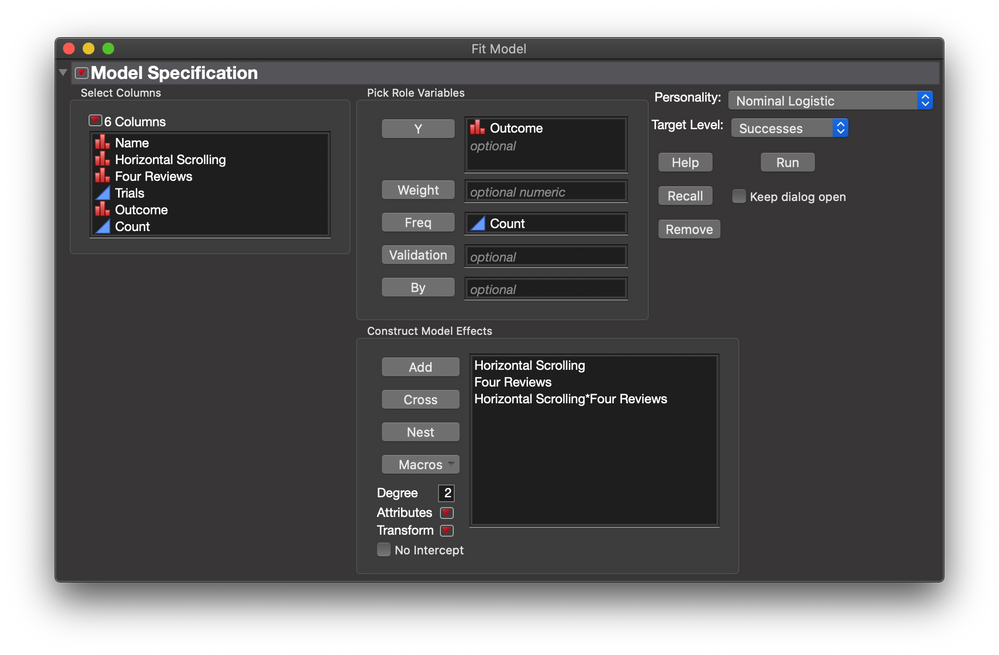
I left the nominal logistic regression choice for the fitting personality but at this point you could select GenReg or GLM with the binomial distribution and the login link function.