Hello everyone,
I have recently attended several courses on Mixture Design. I am now ready to try the principles out in real-life scenarios.
I have the following case. There will be the following things:
3 mixture factors
3 Categorical factors
Most of the recipe is fixed at 83,275% which I will call the BASE - this part will not be changed.
So I have used the Mixture Sum function and set that to the remaining 16,725% (which will be the space for the 3-4 mixture factors)
However, this starting base will have 2 different enzymes added (1 and 2) - a categorical factor 1 that will be made in big batches - meaning this will be a hard-to-change factor.
Those things I know how to do in JMP.
My issue or question relates more to how to combine and select the right terms for my model when combining the categorical ingredient type factors with the corresponding mixture factors.
Categorial factor 2: Stabilizer type (3 levels)
Categorial factor 3: Fiber type (2 levels)
I then have 3 mixture factors:
Mixture 1: Stabilizer conc. (expected to affect the responses)
Mixture 2: Fiber conc. (expected to affect the responses)
Mixture 3: Water (do not expect this to do anything - merely a filler)
I would have all the main terms included.
But is it necessary to have all the second-order terms between the categorical factors and mixture factors??
For example, if we don't expect water (more like a filler) to do anything, should that even be included?
Also, we don't expect Fibers and Stabilizers to affect each other.
This would be my best guess for a model: see the attached screenshot from JMP.
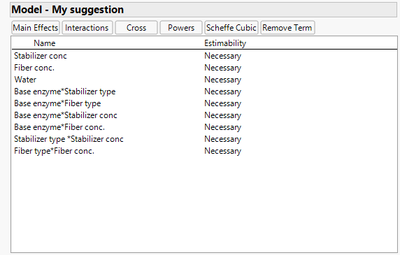
Should other terms be included or??
I would like some input on this. My overall goal is to make some predictions. So my thought was to use an I-optimal design to get as low a prediction variance as possible. However, as a first step, it would also be cool/beneficial if the design could somehow "screen" which of the stabilizers or fibers work best - so they could be excluded going forward. Would a D-optimal design be better for this???
For the first trial my maximum amount of runs i 14. But more could be added later (augmenting).
The JMP file is also attached below.
Looking forward to hearing your thoughts. I am not capable of using JSL or scripting in general.
Thank you in advance.
Best regards
Kristian Bertelsen
Kristian Bertelsen
Technology Specialist