Yes, I would model the mean pH versus the temperature. Yes, the optimum design for a linear response uses just two factor levels.
Be sure that the temperature range is wide enough to produce a large change in the pH but not so wide as to change the nature of the phenomenon you are studying. The point of the design is the modeling. You might think in terms of a narrow range in which you obtain the desired pH. You should think in terms of large change in the response produced by a wider range of temperature. This kind of design will give you more precise and more stable estimates of the trend that you want. This case is experimenting and modeling, not just testing. This case is predicting, not 'pick the winner.'
The last point goes to your final question. We talk about the power in a design. The power is the probability that you will find a real effect (i.e., linear trend) to be statistically significant. Let's say that there is a real linear trend but due to a small sample size you often do not find it to be significant. Such outcomes are false negative results. Power is about the opposite: true positives. The statistical decision in a hypothesis test could be described as deciding if the change in the response is much greater than the change due to random variation in the response.
JMP provides a power analysis built into the design platform. I will illustrate how to use it with an example. I open DOE > Custom Design, change the response name to pH and the goal to None. I add a single continuous factor Temperature with a range -1 to +1 (I don't know the actual range). I click Continue and accept the default model for a linear response to Temperature. I decided to start with 8 runs (user defined) and click Make Design. How will this design perform?
Let's say that when the Temperature does not vary that the pH varies as a normal distribution with a standard deviation of 0.2 pH. Let's also say that we anticipate that the pH will minimally change from 6.4 to 7.0 over the range of Temperature in this design. We are now ready to analyze the power in this design.
Open the Power Analysis outline that is within the Design Evaluation outline. There are two equivalent ways to analyze the power. I will show you both of them. First, enter 0.2 for the Anticipated RMSE (response standard deviation). Now, either enter the Anticipated Coefficients or the Anticipated Response. The Anticipated Coefficient is the effect divided by 2. We expect a minimal effect of 7.0 - 6.4 = 0.6 pH so the anticipated coefficient is 0.3. Alternatively, enter 6.4 as the Anticipated Response whenever Temperature = -1 and 7.0 when Temperature = +1. Click either one of the Apply Changes buttons after entering this information.
The custom design should look like this:
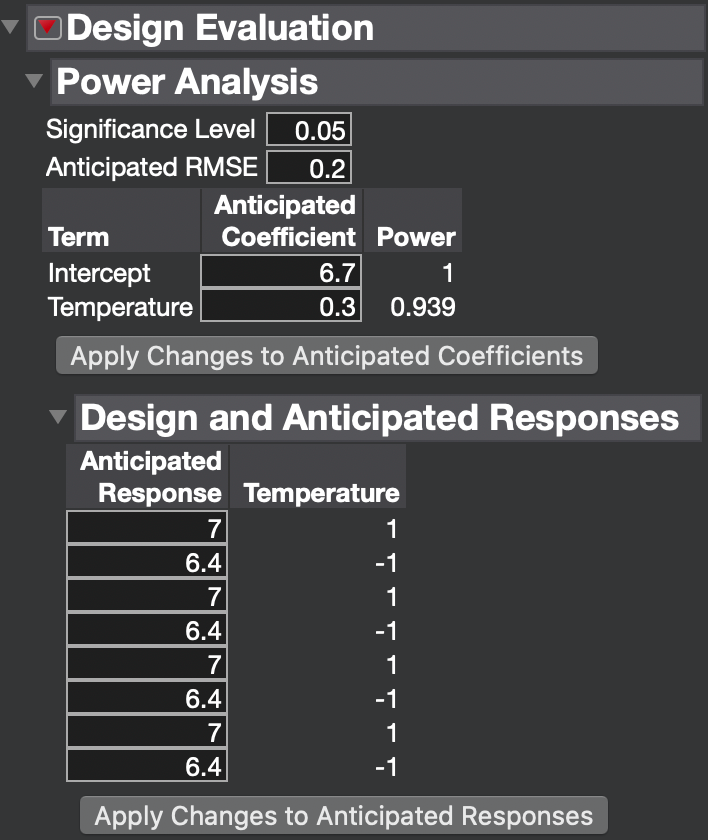
If your expectations are right, then the power is 0.939 and you should find an effect of 0.6 pH or greater to be statistically significant practically all the time using this design.
I made up numbers for this example. Try to translate the illustration into your specific case and see how it goes. Of course, let us know how it goes or if you have further questions.